This eBook presents academic ultra-clean scientific environments and the corresponding digital transformation challenges of these environments, especially the computer science challenges to provide enhanced scientific data integrity
In Section 1, we describe the specificity of academic ultra-clean environments with their requirements and the role of computer science to meet these requirements.
Throughout Section 2, we discuss the digital scientific data acquisition from scientific instruments and their processing challenges for the computing infrastructure.
Section 3 presents the core of the computing, networking, and sensing infrastructures’ challenges to sense, process, distribute and visualize scientific data with high data integrity.
Presented in Section 4 are the difficult sustainability challenges that academic ultra-clean environments must face. The article concludes with a summary of issues that must be solved to speed up scientific innovation and give scientists digital tools to gain further scientific insights.
1. Specificity of Academic Ultra-Clean Environments
Semiconductor chip manufacturing has largely served as the backbone of the digital era. With each new generation of computing, (calculators, computers, smartphones, AR/VR glasses), the supporting hardware has evolved and innovated throughout the years to achieve the performance and cost requirements necessary to make handheld computing devices a reality and ubiquitous among modern society. An example of one of the innovations required for chip manufacturing is the adoption of ultra-clean environments such as cleanrooms, as shown in Figure 1. As the size of chips and the sensitivity of processing these chips became more stringent, the use of modern cleanrooms needed higher control of the environment.
The reason is to prevent stray particles from affecting chip yield and to create a controlled environment that provides stable humidity, temperature, and airflow to significantly improve chip yield and device performance.
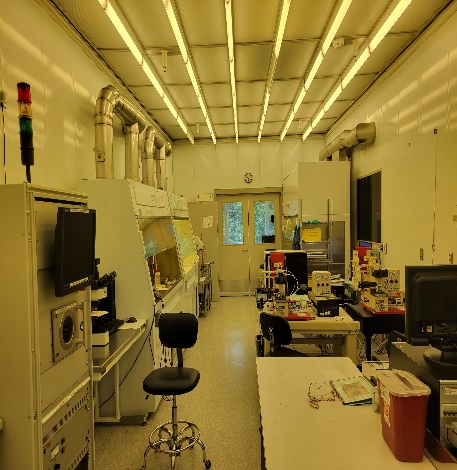
Silicon is the most widely used semiconductor material for modern chip manufacturing. Each new generation of integrated circuit performance improved in speed and capability every two years (a cadence commonly referred to as Moore’s Law) by shrinking the size and consequently increasing the density of transistor chips. This trend continues even today when the average size of a transistor has reached the level of single nanometers. For perspective, the diameter of a single strand of human hair spans approximately 25,400 nanometers. As a result, if a single strand of hair landed on a wafer, thousands of devices would be wiped out due to processing failures caused by the human hair. This exemplifies the strict cleanliness required of cleanrooms to manufacture modern semiconductor chips.
Academic cleanrooms and their equipment at universities are very different from industrial cleanrooms. These differences stem from the fundamental functionalities that each is required to support. In industrial cleanrooms, these ultra- clean environments are designed to facilitate high-volume, high-yield manufacturing. With the supporting capital of multi-billion-dollar companies (Intel, TSMC, Samsung, etc.), these cleanrooms are equipped with state-of-the-art equipment and sensors with the mission to produce the same chip design in massive quantities. Industrial cleanrooms are equipped with the highest degree of cleanliness and a sensory network that constantly monitors and provides a strict controlled clean environment. Chip manufacturing involves hundreds of processing steps that must be strictly controlled to achieve functioning integrated circuits. Since industrial chip manufacturing produces the same process repeatedly, chip manufacturers can collect a large batch of read-out data from each process. Read-out data such as temperature, pressure, and plasma power can give indications as to the “health” of each process.
On the other hand, academic cleanrooms function as a testbed to explore and investigate riskier innovative ideas. As a result, research topics such as quantum computing, 2D materials, and flexible electronics tend to introduce more exotic materials not commonly seen in an industrial cleanroom. These other materials often require a different set of fabrication chemicals and safety standards that a silicon chip cleanroom would not typically encounter. In addition to the materials that are introduced, the personnel of cleanroom users are quite different as well. In an industrial cleanroom, there are manufacturing teams with supervisors, engineers, and technicians that form a well-trained group with the single goal of manufacturing chips in a cleanroom. However, in an academic cleanroom, the users are mostly graduate or post-doctoral students that do not receive the same calibre of intensive cleanroom training. Furthermore, the goals and research of each student are vastly different from one another. This requires a cleanroom capable of supporting research of diverse materials and devices that is also used largely by younger and less experienced personnel compared to industrial cleanrooms. As most academic cleanrooms do not receive the same capital investment as industrial cleanrooms, most of the equipment and sensory networks are old and outdated. It is therefore important for digital transformation researchers to develop low-cost, self-deployable sensory networks that achieve the same functionality as the large expensive sensory networks of industrial cleanrooms to continue producing competitive and innovative research.
Challenges of Academic Cleanrooms:
Most equipment used in academic environments as scientific tools were designed for industrial fabrication applications. Thus, although these scientific tools can be used for a variety of use-cases, their ideal state is to repeatedly run a single process allowing for easily monitored tool health. In academia, however, these tools are pushed to their limits. Each tool will be used for a large diversity of processes by a variety of users who may have minimal experience with the tools. With limited budgets, academic cleanrooms tend to have older, manual tools further exacerbating the difficulty of maintaining the systems and will rarely have backup equipment for when the tools inevitably need to be fixed. The goal then for academic cleanrooms is then robust observations of the tools so preventative maintenance can be performed, limiting the downtime of these expensive, essential tools.
The greatest challenge with academic cleanrooms and research is to support very diverse processes with limited digital datasets. The processes in an academic cleanroom are expensive due to the low-volume and customized nature of the research. This leads to the vastly lower number of digital measurements produced in an academic cleanroom that is needed for artificial intelligence and machine learning (AI/ML) algorithms to achieve high accuracy data classification and/or object detection. Furthermore, most academic cleanrooms are equipped with outdated equipment and do not possess a sensory network for environmental monitoring around equipment as industrial cleanrooms do due to the level of the cost required to implement these features. The capability to deploy low-cost sensory networks that implement preventive maintenance in an academic cleanroom is therefore important to sustain a cleanroom environment that is competitive with state-of- the-art technology for academic researchers.
2. Scientific Data Acquisition and Processing from Scientific Instruments
For semiconductor processing, a large variety of digital data is produced during the scientific process. Datasets that include processing equipment read-out such as gas flows, plasma power, and pressure provide a measure of the process characteristic (deposition thickness, etching depth, etc.) as well as process consistency and equipment health. On the other hand, several critical steps during the device processing may require additional measurements to guarantee the accuracy and precision of the process. For instance, Scanning Electron Microscopy (SEM) images are used to verify sidewall profiles of etching processes. The main challenge is that each process can require a different set of equipment and a different set of measurement tools to verify that process. For example, while in the case of etching, the equipment was an ICP-RIE etcher and the verification tool was an SEM, in the case of deposition, the equipment is a PECVD whereas the verification tool is an ellipsometer that measures film thickness.
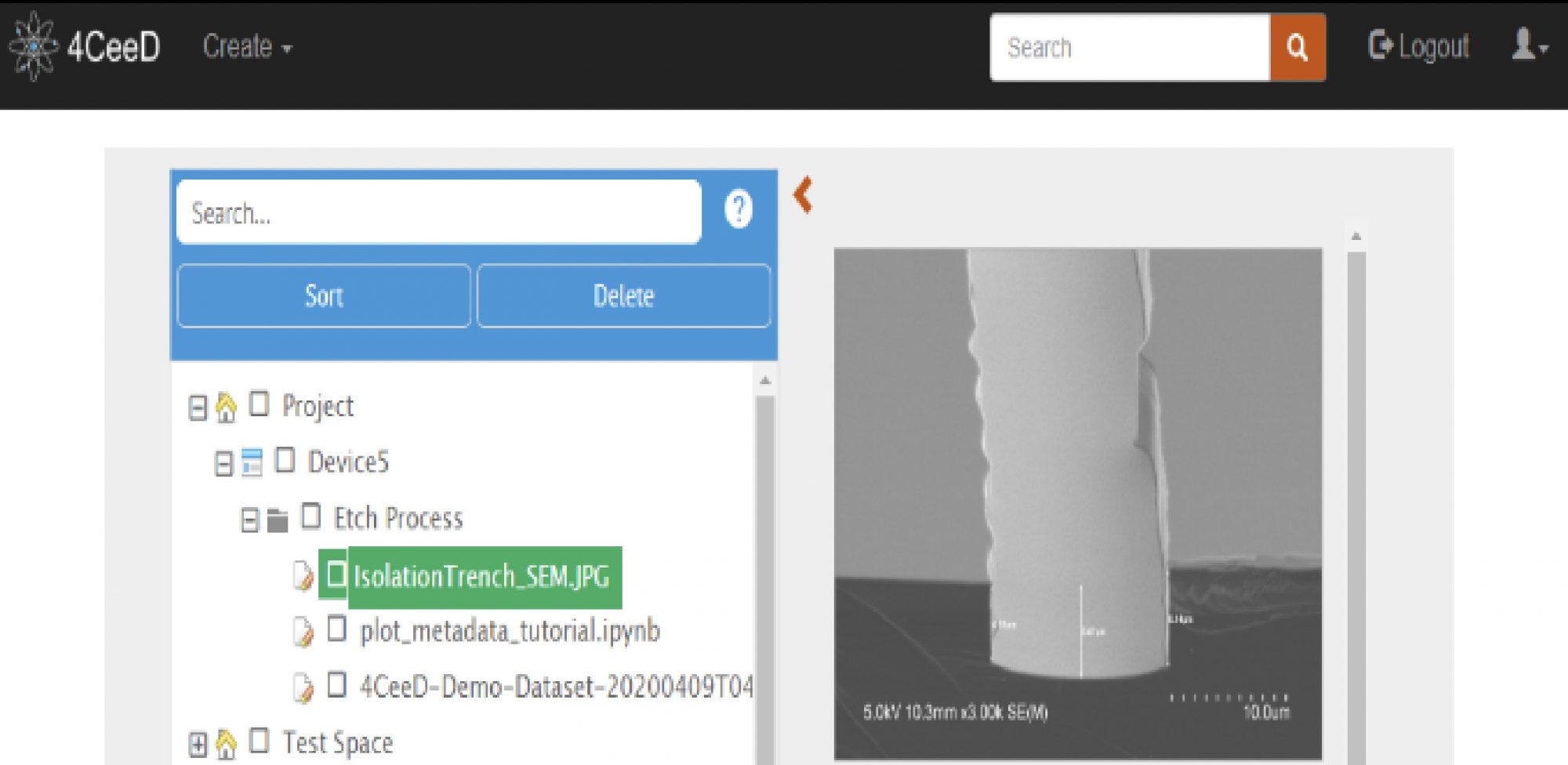
Given the wide variety of tools and their inconsistent usage from one academic researcher to another, the data collection process is often very manual. For items like process parameters and outcomes, such as the mentioned example of etching with parameters such as gas flow or power and characteristics such as etch depth, a range of manual note-taking techniques are used at the time of the process. Most common methods include writing notes in individual notebooks or inputting notes into individual or shared documents stored online. For other datasets like images from a microscope, e.g., SEM (see Figure 2), where the data is already digitized, these are collected through shared drives, specifically designed scientific data storage systems, or local USB storage devices if internet connectivity to the microscope is not present due to tool age and security concerns. Most processing of this data is then done in separate labs or offices after the cleanroom processes have been done.
Challenges of Scientific Data Acquisition and Processing Workflows
The challenges of scientific data acquisition and processing include (1) data curation and processing, (2) multi-modal data fusion and (3) failure analysis.
Data curation and processing:
Due to the diverse dataset that is accumulated over an entire device creation process, and the lack of a centralized data infrastructure that automatically combines the datasets from each tool into a central location, most academic cleanroom data is very isolated and discrete. While in principle, the collection of data is interlinked because each process is serially conducted and impacts the process after it, for academic researchers, most data is separated and often does not contain the proper process information describing the previous processes that have accumulated to the resulting dataset. For instance, if there are 6 process steps conducted before a researcher takes an SEM image of the fabricated device and realizes there is an error, the researcher does not know if it was step 5 or step 1 that is the root cause of the error. Only with the combined information of each process step can it be fully concluded which step caused the process failure.
Furthermore, the currently existing data storage infrastructure for microscopy images such as file explorer and google cloud are based on a “tree view”. Without tediously opening each file, the “tree view” only allows users to input experimental parameters in the file name. This leads to extremely long file names that serve to encompass the entire experiment in key-value pairs such as “06-10- 2022 GaAsEtch_BCl3-20sccm_Cl2-10sccm_Ar- 5sccm_RIE-200W_ICP-400W_8mT.txt”. We have developed a research system, called 4CeeD is a system [Ngyuen2017] that displays all pertinent information in one easy format that alleviates the issues of using a “tree view” data storage system (See Figure 2). Further integration of 4CeeD to achieve automatic data logging would be the final goal for a desired data storage system. However, challenges arise when digitizing data from old, outdated equipment that still uses analogue readout panels while also navigating through the proprietary software control systems of new fabrication equipment. An open-source method of interfacing with processing equipment tools is required to fully develop a low-cost, centralized private cloud data storage infrastructure that automatically collects data from each piece of equipment for academic researchers.
Multi-modal data fusion:
The main challenge with collecting data from a cleanroom fabrication process is the diversity of data that is produced from a wide variety of scientific equipment. Furthermore, the interlinking and cascading effects of each process make each dataset a representative of multi-modal data fusion. The challenge is how to automate tracking of the whole process, and interlink and correlate data.
From an individual fabrication process perspective, each process can have multiple datasets that describe the same phenomenon. For instance, a lithography process will have the lithography recipe with key-value pairs that describe the spin speed that the photoresist is dispensed, the exposure dosage that the photoresist is activated for, and the development time that the unwanted photoresist is washed away. However, to verify the success of this process, an optical or SEM image is taken of the top-view and sidewall view to verify and ensure that the correct dimensions and sidewall profile are successfully replicated.
Then from an interlinking process perspective, each process characteristic is propagated through the next process. For instance, etching is a common process followed by lithography. If there is a defect in the lithography process that is not identified during the visual inspection step, this defect will propagate into the etching process. Once it is identified during the visual inspection after the etching process, a misconception can occur where because the defect was identified during the etching process, a false conclusion that the etching process has an issue can be made. However, the true failure mode occurred during the lithography process. Eliminating false conclusions can save precious material, time, and processing resources that significantly increase productivity in academic as well as industrial cleanrooms.
Failure analysis and anomaly detection:
Failure analysis in fabrication processes is often done manually via visual inspection to track the consistency and desired features of microscope image datasets produced during the fabrication process (see Figure 3 for SEM images from successful controlled experiments and failed experiments). For instance, in lithography steps as aforementioned, there is a visual inspection step that occurs to ensure the desired outcome of the lithography process is met. However, these inspections are rather qualitative from an academic user perspective. Whether or not the shape, sharpness of the edge, and colour of the photoresist look “correct” is up to the user. Using AI/ML, a quantitative method to determine whether the photoresist will yield a successful or unsuccessful process is an extremely powerful tool [Wang2021].
Furthermore, introducing additional process variants and observing the effect may lead (1) to a tool that can be used to predict the overall photolithography process result without wasting the resources and (2) to an experiment that can be extremely helpful for academic researchers and industry professionals.
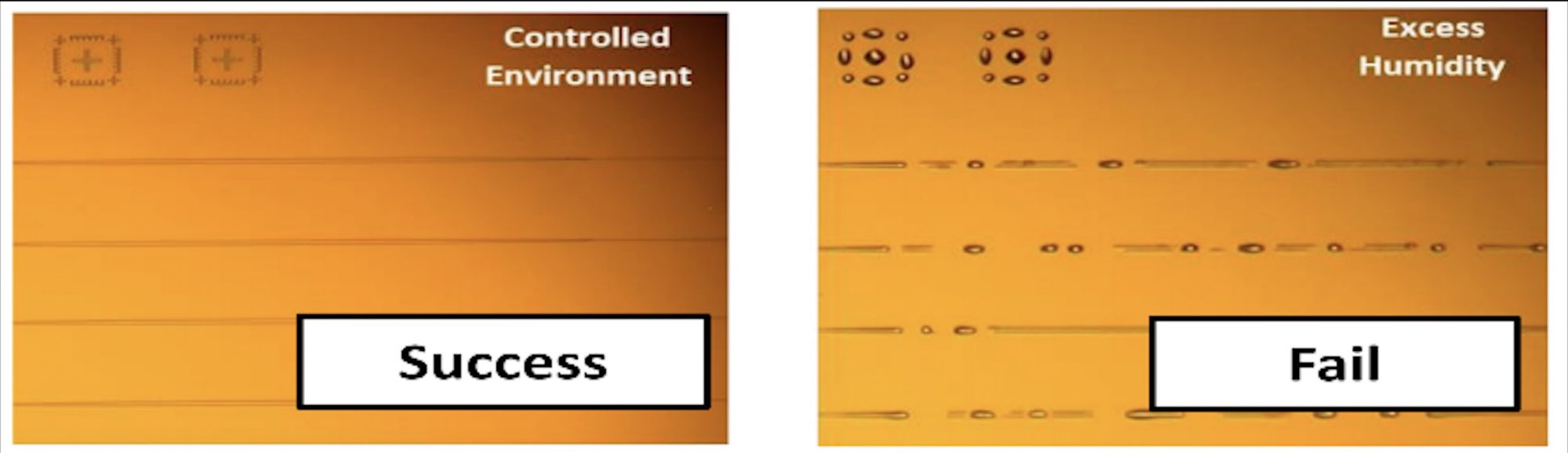
However, the main issue is the lack of microscope image data sets that are produced in an academic cleanroom setting. Due to the lower volume and more custom processes academic cleanrooms produce, the datasets are very small and are very diverse from one another. This leads to challenges when creating an AI/ML training algorithm to determine whether a fabrication process is a success or a failures.
Another challenge concerning anomaly detection is the lack of ground truth labels for the sensory data deployed externally in cleanrooms. The large-scale sensory data (e.g., humidity, temperature, vibration sensory data) collected from the various sensors placed around the cleanroom equipment and from digital communication processes change rapidly over time and are bound to be noisy. The anomalies contained within this data are often characterized by subtle process deviations. These anomalies often get contaminated by the surrounding noise that may overshadow the few, rare anomalous events. Thus, annotating these data values with the correct labels is notoriously difficult. The absence of these ground truth labels makes the AI/ML-based anomaly detection process rather more challenging, resulting in high false positives rate or high false negatives rate due to the dominance of spurious anomalies. Thus, collecting the data and labelling it in the wild is imperative to correctly identify the realistic anomalies and to ensure the robustness of the AI/ML-based anomaly detection algorithms.
3. Sensing and Computing Infrastructure for Academic Ultra-Clean Environments
The need for automation and digital transformation in the semiconductor industry influences the design and building of cyber- physical systems infrastructure within cleanrooms to create new digital services. The sensing and computing (sense-compute) infrastructure [Nahrstedt 2020, Tian2021] within these academic cleanrooms consists of a networked system with three components: sensors, edge devices, and cloud (see Figure 4). The main objective of the sense-compute infrastructure is to observe, maintain, and improve the performance of the physical equipment (e.g., fume hood shown in Figure 4), fabrication processes and machinery within cleanrooms.
To achieve the objective, we briefly describe the sense-compute infrastructure in Figure 4. First, the infrastructure aquires, distributes, processes, analyses, visualises and reasons about SEM images, or external sensors (e.g., humidity, temperature, vibration, sound).
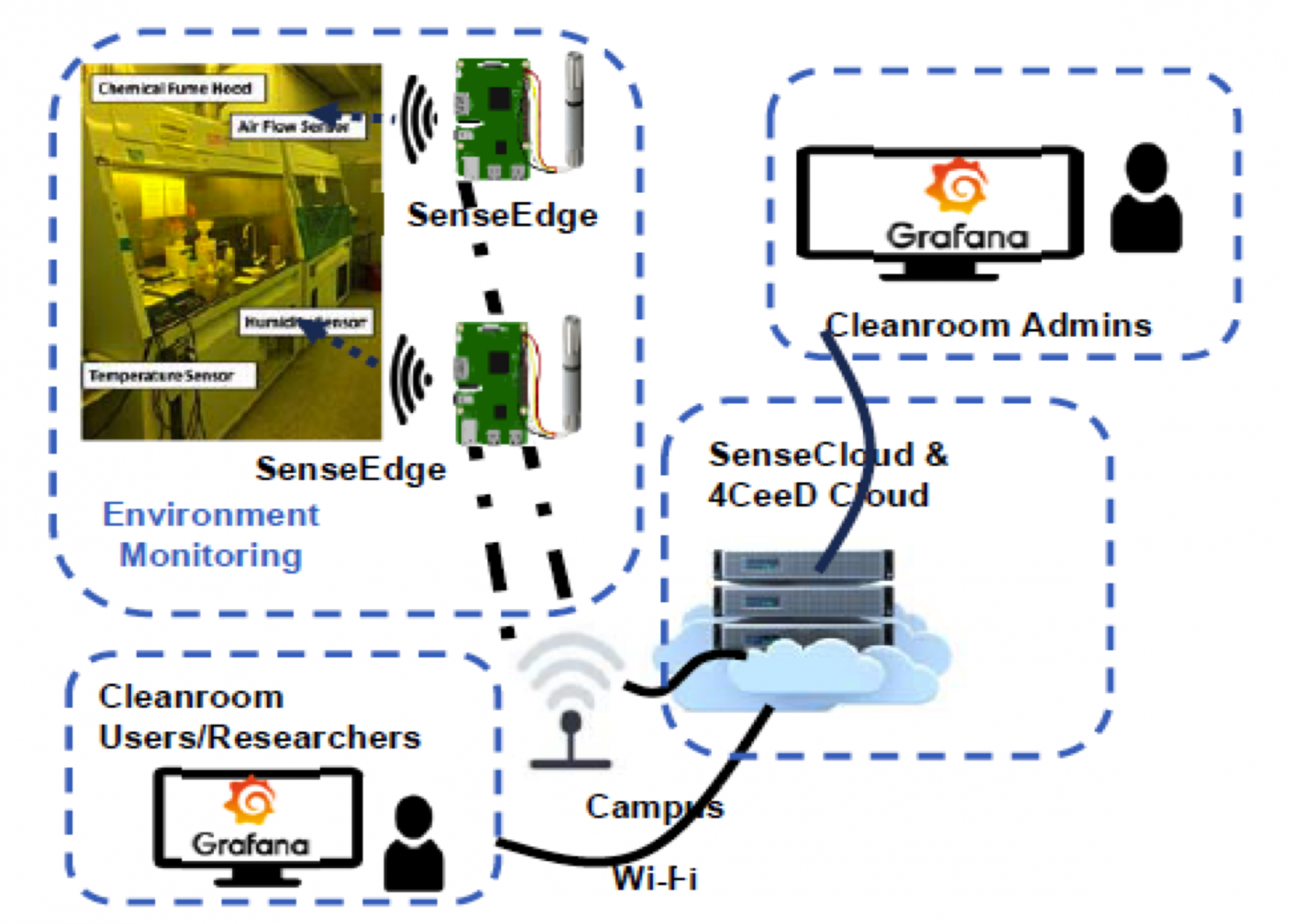
Second, the sense-compute infrastructure is used for self-monitoring of equipment operations and the surrounding environment by sharing continuous data from sensors and providing real-time visibility to the cleanroom administrators and researchers. Third, sensors convert various physical parameters into electrical signals and micro-controllers convert electrical signals into digital data, preprocess and offload data to edge devices (e.g., SenseEdge) or private cloud servers (e.g., SenseCloud, 4CeeD Cloud in Figure 4) via network infrastructure [Tian2021]. Forth static information from the machine’s manual documentation and repair instructions in addition to the real-time sensor data values is used for detecting failures in cleanroom machinery. Fifth, AI/ML-based computational capabilities such as anomaly detection, failure prediction, and visualization dashboards are used for identifying the wear and tear of the machines and for diagnosing faults and failures. Data mining and real-time analytics in the cloud help reduce the maintenance cost by providing scalability and usability among various applications. Finally, all sensory data, equipment data, and results from data analytics get collected and stored at edge devices and private clouds.
Challenges of Sense-compute Infrastructure
To find out more, click the accompanying eBook course of an experimental procedure with erroneous readings, which can be extremely costly. The sense-compute infrastructure driven by the cleanrooms’ high-end equipment is expected to be well-operated in multiple complex scenarios from identifying fine-tuned anomalies to making recommendations in near real-time. Thus, guaranteeing a seamless performance of the sense-compute infrastructure within cleanrooms can pose several fundamental challenges.
Infrastructure failures and cascading effects:
The infrastructure is a networked system of various sensors and edge computing devices communicating data to a private cloud in real- time. This strongly interdependent infrastructure with system-wide connectivity is vulnerable to various types of failures due to network partitions, unexpected server restarts, power outages, out-of-memory database errors, and frequent software upgrades. These failures can lead to cascading effects on other integrated components of the system causing an eventual collapse of the entire computing infrastructure. For example, failures in one of the edge devices can cause failures of the dependent sensors and nodes transmitting data to that edge device, which can lead to loss of data and subsequent service shutdown in the cloud.
Resource-constrained devices:
Meeting the computational requirements of AI/ML-based services for anomaly detection, failure prediction, and multi-modal data analytics with limited in-situ resources (memory, battery, processing power) for sensors and edge devices is a critical challenge. Typically, cloud servers are located distant from the physical sensors and edge devices which introduce the problem of large latency for offloading the computations to the cloud. Additionally, the transmission of large amounts of data (including multimedia data such as images, audio, and video streams) to and from the cloud server raises issues due to limited bandwidth. Therefore, it is important to alleviate the resource-constrained problem by offloading computation-intensive tasks closer to the sensors and edge devices to ensure low latency without trading off the performance of the AI/ML-based algorithms.
The scale of sensors:
The scale of sensors is typically large in sense- compute infrastructure because we need to deploy sensing devices in cleanrooms and buildings. The scale causes an increase in cost, deployment, maintenance, and network bandwidth load.
Sensory data heterogeneity:
Heterogeneous modalities of data are produced by different types of sensors, edge computing hardware and embedded software within cleanrooms. Varied modalities of data include time-series data, audio data, vibration data, events, control signals, network traces, images, and video streams. The data is collected and transmitted in different formats, frequencies, and granularities by different sensors, which makes it difficult for the existing tools and algorithms to analyze them. The availability of heterogeneous data from multiple integrated sources requires intense data cleaning mechanisms specific to each type of data before storing and handling them to make meaningful insights.
Furthermore, heterogeneous sensors require different connectors, supply voltage, and communication protocols. Integration of heterogeneous devices and collection of heterogeneous sensory data is challenging. Our previous work SENSELET++ [Tian2021] started the path of new uniform interfaces for heterogeneous sensors. However, due to new sensing modalities, the interfaces need to be regularly updated to accommodate new sensors.
Cleanrooms introduce electrical and magnetic interference and noisy situations. These situations impact the integrity of the deployed sensors and the quality of the sensory data. The noise interference then reduces the performance and connectivity of sensors. For example, the background noise greatly reduces the performance of microphone arrays which are used to ‘hear’ abnormal behaviour of cleanroom instruments. The challenge is to isolate interference sources and restore the integrity of sensory data.
Lack of standardization:
The unified sense-compute infrastructure for cleanrooms consists of tightly coupled sensors, communication protocols and edge devices, which are often supported by the legacy hardware, proprietary protocols and software stack (embedded operating system). The integration of these legacy systems and private protocols is a challenge as these systems do not agree on one common model for backend tasks including processing, storage, firmware updates and patches. Thus, the interoperability between this multitude of protocols and software/hardware standards results in isolated systems with siloed data warehouses and security gaps. To address this, an integrated platform that supports different types of devices, public protocols, and operating systems is required to ensure better interoperability, portability, and manageability of cleanrooms’ sense compute infrastructure.
Cloud reliability:
Cloud serves as the centralized system for data storage and sharing across multiple applications and services. This can be either a public cloud compute or storage offerings such as Amazon’s AWS or Microsoft Azure, or a private cloud solution. However, the centralized data storage infrastructure could present a limitation in the form of a single point of failure if proper redundancy and resiliency are not used, causing loss of all the data and poor system performance. As the primary output of a sense- compute infrastructure is the data, data storage resiliency must be paramount for the success of such a project. Replication and distribution of database services using something akin to MongoDB Replica Sets and replicated and distributed filesystems would be the first step to ensuring high availability of the central storage. However, regular backups with at least one copy held off-site would be ideal to protect against hardware failure as well as facility failure such as a compromise in the cooling of a data centre.
Edge device design:
Edge devices are an integral part of the sense- compute infrastructure in cleanrooms [Yang2019, Nahrstedt2020] as shown in Figure 4 and their design and implementation must be carefully considered. First, the edge devices should be highly customizable. This requires that the edge devices support the storage, management, processing, and transmission of various data types. Different network stack protocols corresponding to these applications need to be supported. In these regards, the edge devices need the ability to be customized by clean room infrastructure managers. Second, the edge devices need a layered design. Application layer protocols need to be implemented agnostic of the underlying edge device hardware. Different layers need to be designed independent of each other and communicate with each other with Application Programming Interfaces APIs. In this way, in the future when any layer of these devices – including the hardware layer – needs to be upgraded, it can be upgraded gracefully without impacting a lot on other layers. And third, the edge devices need to be reliable and available. Since the edge devices support various applications, some applications are critical to the lab environment, such as monitoring water leakage. For these applications, data need to be reliably collected and analyzed at the edge devices and they need to be highly available to lab managers.
Security and privacy threats:
Security should be enforced on the sense- compute infrastructure of the academic clean rooms throughout its development and operational life cycle. The infrastructure is vulnerable to various types of attacks at different levels such as the sensor data manipulation attack, Man-in-the-Middle attacks, Distributed Denial of Service (DDoS) attacks, replay attacks and node hijacking. To combat these threats, various security principles such as data confidentiality, software authentication, authorization and data integrity should be taken into consideration for securing the end-to-end infrastructure.
User privacy can be an issue when sensory data come from auditory and camera sensors. The challenge is to localize the sources, anonymize, and remove private information, i.e., leave only relevant data that contribute to the integrity of the cleanroom scientific process.
Safety and reliability:
The close interactions between the cleanrooms’ sense-compute infrastructure and the surrounding environment present a new set of safety hazards. As most of the sensors and edge computing devices are deployed “in the wild” within the cleanrooms, they are exposed to physical damage during accidents or physical attacks. For example, during an untoward event such as leakage of toxic gas, fire or a pipeline explosion in clean-rooms, damage can happen to both the physical and cyber (sense-compute) components of the infrastructure in close vicinity or distant from the point of incidence. This can cause system degradation and potential harm to humans. In the event of such unexpected hazards, the automated safety functionality and notification service of the sense-compute infrastructure will also be disabled. Therefore, failing to foresee such events can impact the reliability as well as safety of the infrastructure. This makes it imperative to have a comprehensive evaluation of the robustness of the sense-compute infrastructure under various abnormal environmental conditions. Additional physical safety barriers may be required to prevent damage to the sense-compute infrastructure deployed within the academic cleanrooms.
Challenges of Digital Twins
In addition to the core components of the sense- compute infrastructure, data-driven digital twins are designed and deployed within cleanrooms to support performance optimization of the equipment and communication processes. These digital twins are the virtual representations of the physical entity that provide both structural and operational views of how the physical system behaves in near real-time as shown in Figure 5. This allows the cleanroom administrators and researchers to monitor the system dynamically and emulate “what-if” scenarios on the virtual twins before applying those changes to the physical object in real-time. The digital twin representations pose several challenges:
Reliability of data-driven models:
A primary challenge in designing virtual replicas of the cleanroom equipment is the need for mathematical models and physics-based simulation knowledge of the underlying mechanics, which is infeasible due to inaccessibility to the manufacturing design details of the legacy systems. Therefore, to counter this, data-driven digital twins are designed to replicate the behaviour of physical equipment in near real-time. For performance guarantees of these digital twins, reliable data-driven models are required to capture the behavioural dynamics of the system to make accurate predictions.
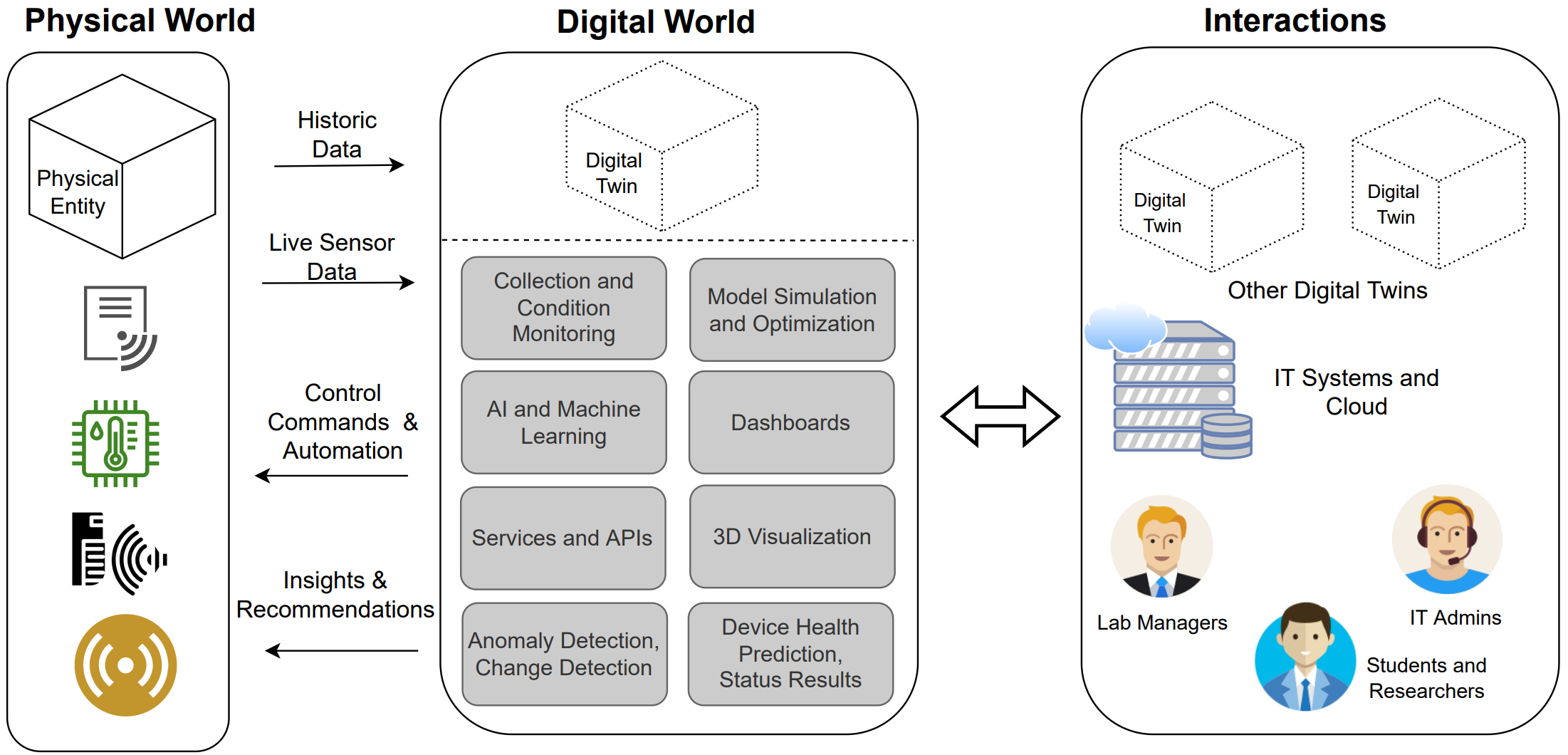
The consensus among multiple digital twins:
A networked system of multiple digital twins may exist in the sense-compute infrastructure as shown in Figure 6. Each digital twin will perceive and model the behaviour of the actual physical component, analyze a large amount of data, and make predictions and recommendations for control decisions and strategic maintenance. However, the control decisions and recommendations made by each digital twin can be conflicting with one another as they will be derived using the respective local sensor data. These conflicts in the decision- making process of the digital twins can become more prominent when there are faulty sensors, malicious sensor behaviour (e.g., Byzantine faults) or noisy measurements. This can disrupt the unity of the network and lead to erroneous decisions, predictions, and recommendations. Therefore, distributed consensus algorithms are required on top of the networked digital twins, to make decisions based on collaborative filtering and contextual information in addition to the local sensor data.
Communication delay:
Communication delays can have a severe impact on the performance of the digital twins. Due to the dynamic nature of the sense-compute infrastructure of the clean rooms, large latency between the physical equipment and virtual twins can be a major bottleneck. The digital twins may fail to accurately reflect the current/up-to-date state of the physical infrastructure, and the predictions made by the digital twins may become obsolete in the presence of the rapidly changing state of the communication network and the cyber-physical systems within cleanrooms.
Challenges of Sense-Compute Networks:
Most academic cleanrooms reuse the university campus network infrastructure including the wireless WiFi and wired Ethernet networks. The campus network is well designed for providing security features that can protect against external network attacks. The network is also maintained by a specific university IT (Information Technology) team which guarantees reliability. However, the sense- compute infrastructure may face network performance and availability challenges when using the wireless network. Specifically, wireless sensory and edge devices might experience slow connectivity or even no connectivity at all. The network performance challenges mainly originate from the fact that the existing network infrastructure (algorithms and hardware) is mismatched with the new requirements that come from the sense- compute infrastructure:
A large number of connections:
The network infrastructure must serve many wireless sensory and edge devices in cleanrooms. However, traditional non-enterprise wireless access points will lose performance when serving too many devices.
Sufficient offloading bandwidth:
The sensing infrastructure consists of diverse sensors such as temperature, humidity, vibration sensors, microphones, and cameras. On the sensor side, there is not much storage and computing capability, hence we need to offload a large amount of data to a remote server (edge or cloud), requiring sufficient offload bandwidth.
Coexistence of wireless technology:
The sensing infrastructure adopts different wireless technologies such as WiFi, and Bluetooth to transmit sensory data. However, these technologies may interfere with each other and may influence the network performance, especially when using 2.4 GHz Wifi which operates on the same frequency as the Bluetooth network. What makes things worse is that the operation of equipment in the cleanroom can also generate such interference. Hence, the network infrastructure should include wired Ethernet connections for high- noise environments, and allow WiFi connections at both 2.4 GHz and 5 GHz.
Transmission of multi-modal data:
Data collected at edge devices belong to various applications, such as scientific instrument data (e.g., SEM images), environmental data (e.g., temperature, humidity data), and equipment maintenance data (e.g., vibration, audio data). These data have various bandwidth requirements, different priorities according to their importance, and different latency requirements.
Reliable network connectivity:
Data streams from the sensing infrastructure are subject to a variety of factors such as available bandwidth, and network congestion which can lead to intermittent packet drops and loss of data. Reliable network connectivity is required that ensures the delivery of the data to the storage infrastructure in the cloud. Additionally, it is important to detect when the sensing components in the infrastructure become offline or when it drops off the network. This will help in continuous monitoring of the state of all networked edge devices, and if any device/sensor goes offline it can help to identify and fix the problems arising due to network connectivity issues.
4. Sustainability
Ultra-clean academic scientific environments, as discussed above, consist of diverse instruments, interconnected with diverse edge-cloud networked infrastructure. These diverse instruments (e.g., microscopes) get purchased and updated at different times and often live in the academic labs for 10 to 20 years serving scientists to measure and acquire data from their experiments. However, as these instruments go through digital transformation and get connected through their digital compute and network components to edge- cloud networked infrastructures on campuses, they are facing a mismatch between the sustainability and updates of scientific instruments (e.g., optics) and the sustainability and updates of the surrounding cyber- infrastructure where they are embedded and connected to. The scientific instruments (hardware and scientific software) may be functional for 10-20 years and do not need to be upgraded or replaced. On the other hand, the cyber-infrastructure with its computing, sensing, and network hardware and software, with its data formats, storage, security, and maintenance needs to be upgraded every 6 months to 1 year to keep up with security patches and new features of the modern IoT-edge-cloud infrastructures. Hence, the goal for sustainability in these environments is to bridge this gap between the ever-changing cyber-infrastructure and the ageing scientific instruments.
Challenges of sustainability:
Sustainability of the cyber-physical instrument and sense-compute infrastructure in cleanrooms faces several challenges:
Operating system security mismatch:
Because of the longitude of the scientific instruments (up to 10-20 years), these instruments may run scientific software tools on old operating systems (e.g., Windows XP, Windows NT). On the other hand, the development of operating systems (OS) is moving fast, and OS systems get upgraded with new security patches and new functionalities every few months. But if system administrators upgrade the instrument’s OS from Windows NT to Windows 10, the scientific software tool will not run because the scientific software tool was built for Windows NT and was not upgraded to Windows 10. Hence, the system administrators leave the old OS at the level where the scientific tool can run, and the scientific instrument can be used.
Academia-industry mismatch:
One may ask why the academic labs do not upgrade their instrument hardware and scientific software tools as fast as the new OS comes on. The reason is cost, the lag between new OS and new scientific tool development, and discontinued support of the instrument hardware and software after a few years. The companies assume that the academic laboratories will replace their equipment. However, academic laboratories cannot afford the replacement due to cost. Hence, they must continue to work with aging equipment to maintain research and educational activities. This gap introduces security loopholes, and the equipment is taken off the network to avoid any remote access and hence any remote attacks on the scientific equipment. Once the equipment is offline, scientists will use USB memory sticks to collect data from the scientific equipment, and move the data to their laptop/server data management system via “Sneakernet”, it means the scientistis will walk with the USB memory stick from the cleanroom to their office computer.
Network Mismatch:
If scientific equipment has too large a technological gap between their scientific software system and the remote data management system in the private cloud, the aging equipment will not be able to keep up with the modern network system in terms of network speed and connectivity. For example, an aging equipment may be able to operate only on 2.4 GHz frequency Wifi, whereas the private cloud operates exclusively on 5 GHz WiFi frequency. This network mismatch causes that the scientific equipment cannot connect to the WiFi at all. In this case either the scientific equipment will function off-line or a custom solution for that scientific instrument must be found.
Non-scientific application software:
Because of the OS security mismatch problem, various non-scientific applications (e.g., web browsers) will not be upgraded, introducing another level of security and functionality difficulties. For example, web browsers are developed together with web-servers. It means that if a web-browser cannot be upgraded, the web-server needs to stay the same, causing difficulties of maintenance and security at the remote cloud side, and requiring extra steps of protection such as sand-boxing of the older web-server into a virtual machine or docker environment.
USB sticks problem:
As mentioned above, many of the aging scientific equipment will be taken off-line if the OS and the corresponding scientific software tool cannot be upgraded. In this case, the scientists will use USB memory sticks to get the data from the equipment’s computer and use ‘Sneakernet’ to walk/copy the USB memory stick to their laptop/server data management. However, USB memory sticks represent security risks because an attacker can put malware on the USB memory stick and once the USB memory stick is put into the equipment computer, it can damage the older scientific software, making the scientific equipment itself unusable or even damage the equipment. Another challenge with USB memory sticks is their limited storage. Because of the limited storage, a scientist needs to first spend an extensive amount of time on the equipment computer to (1) filter out unwanted raw data, (2) convert data from raw data format to a transferable compressed data format, and (3) fit the compressed data onto the USB memory stick. These digital transformation operations take time, often half of the reserved time on the scientific equipment, which tremendously decreases the productivity of the equipment, and increases the cost of usage of the equipment [Ngyuen2019]. Note that each user pays for the usage of a scientific equipment, hence the goal is to spend most of the time on the actual scientific experiment and minimal time on any digital transformation of the data such as getting it stored in a remote data management system.
Budget limits:
The budget for upgrading academic cleanrooms is very limited. Since sensors are one of the basic units of the sense-compute infrastructure, they should have a low price for large-scale deployment. However, many specialized sensors are costly. For example, industry-level vibration sensors are in the range of several hundred dollars due to designated data acquisition hardware and software. Budget limits also impact the maintenance cost, e.g., sensor repair and replacement, battery replacement, sense-compute infrastructure hardware/software upgrades, and equipment replacement. Therefore, new maintenance solutions need to be explored that take the budget limits into account.
Conclusion
Cleanrooms represent important scientific environments for the semiconductor fabrication area, and academic cleanrooms are especially of great importance to research and fabricate new devices as well as educate new workforces in this challenging area. Digital transformation of these environments is coming to speed up the transformation from data to knowledge and to speed up discoveries and insights from multi- modal data. Hence, computer science and engineering have a major role to play to make this digital transformation happen. However, as discussed above, there are numerous challenges, especially for the academic ultra-clean scientific environments, that need to be solved with innovative, cost-efficient, and performance-efficient sense- compute infrastructure and its hardware/software services. In addition, one also needs to keep in mind the sustainability of this sense-compute infrastructure and new approaches need to be developed to:
(1) Preserve the scientific information over long periods of time;
(2) Bridge the gap between the long-lived physical systems and short-lived cyber-infrastructures,
(3) Enable trustworthy infrastructures over long periods