Klara Nahrstedt, Professor and Director of Coordinated Science Laboratory at the University of Illinois, Urbana-Champaign, and her collaborators explore how data-centric cyberinfrastructures in academic ultra-clean scientific laboratories help speed-up next generation inventions
Academic Ultra-Clean Scientific Laboratories (cleanrooms) are highly complex environments consisting of diverse scientific instruments which (a) enable scientists to make discoveries of new materials, new semiconductor devices, and other scientific discoveries very important and crucial for the society; and (b) serve diverse missions in academic environment, ranging from educating students, conducting outreach to public, to serving as a frontier of discoveries.
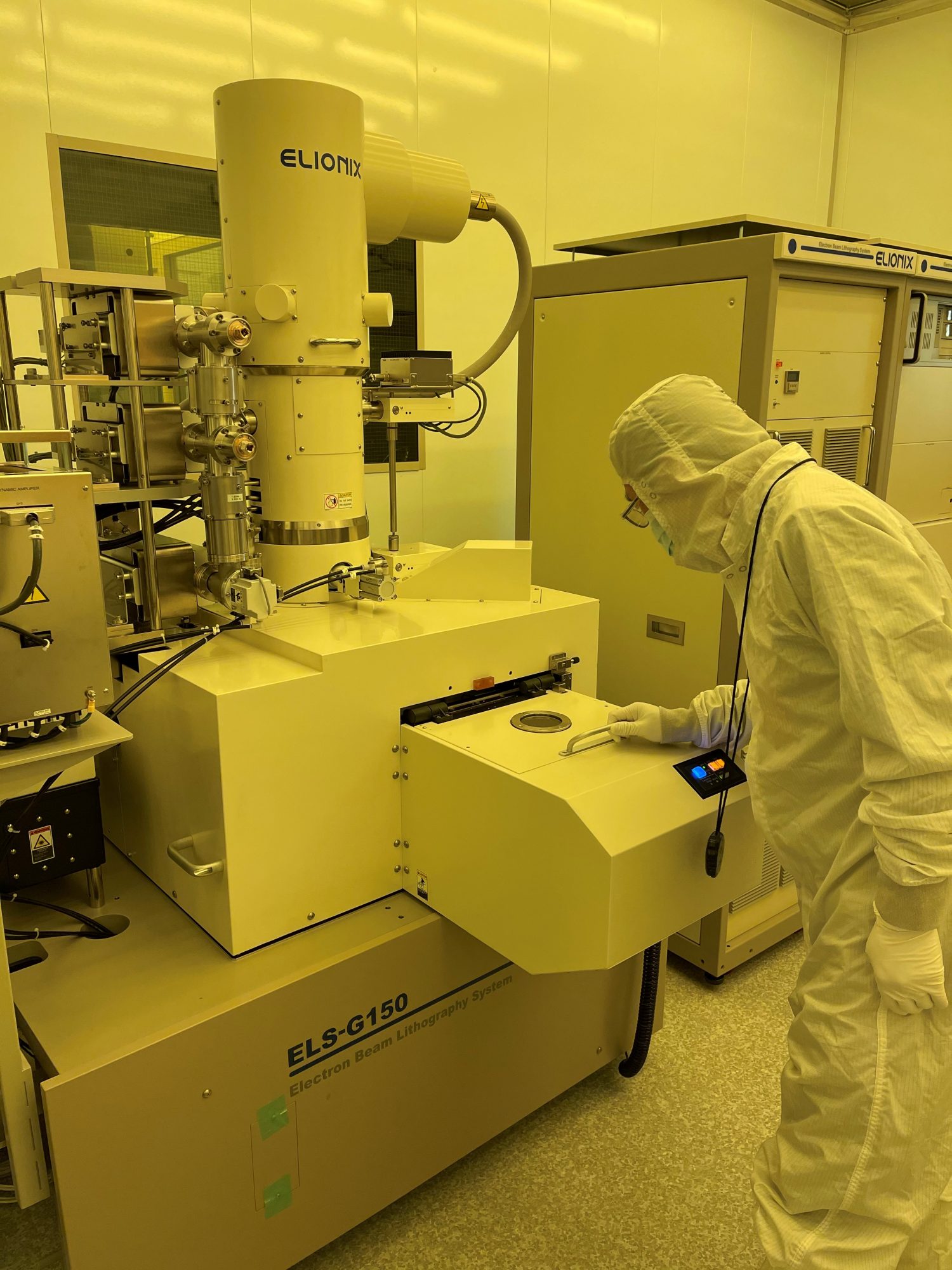
As Figure 1 shows, the scientific instruments are becoming highly digitised, creating, and collecting an immense amount of data locally. But these cleanrooms often lack digital tools and cyberinfrastructures to help with data provenance, searching, and management.
Furthermore, academic cleanrooms lack the capability to provide situational awareness to scientists and lab managers regarding the surrounding space around instruments which can cause failures in experiments, insights, and ultimately in discoveries [1]. Hence, it is of high importance to invest in the development and deployment of data-related tools and cyberinfrastructures to accelerate their scientific discoveries.
Achieving cost-efficient data collection
At the University of Illinois, Urbana-Champaign (UIUC), we research, develop, and deploy data-centric cyberinfrastructures to achieve cost-efficient data collection, processing, tracing, and management in academic environments. Our core data-centric cyberinfrastructure services, discussed below in terms of their functions and insights, are 4CeeD, ProvLet, and SENSELET.
Distributed data-centric cyberinfrastructure (CI) allows timely and trusted curation, coordination and storage of data generated at scientific instruments in cleanrooms such as SEM (Scanning Electron Microscopes) and TEM (Transmission Electron Microscopes). We provide the 4CeeD service [2] for this purpose. Data is uploaded from microscopes into a remote private cloud where it is indexed, stored in MongoDB-based Clowder data management system [3], and prepared for future access, queries, analysis, and visualisation.
4CeeD then allows scientists access to their instruments’ data and metadata via a web-interface. Video in Figure 2 shows the 4CeeD curator and coordinator interfaces during the real-time upload process at the microscope site, and during the visualisation of indexed, queried and analysed datasets.
We gained insights from deploying 4CeeD in the Holonyak Micro-and-Nanotechnology Laboratory (HMNTL) and the Materials Research Lab (MRL) such as how providing trusted and real-time upload capability of scientific data from cleanrooms to private cloud repositories saves time and security headaches, and using open-source software to build data-centric cyberinfrastructures for cleanrooms enables sustainability. This is important since scientific instruments last 10-20 years and software ages much faster. Hence, cyber-components need to be constantly upgraded to keep up with the security and performance demands.
Figure 2: Data-centric Tools for Ultra-Clean Scientific Laboratories
Data provenance for 4CeeD is done by ProvLet to identify and track who created scientific data in cleanrooms, when it was created, and where it was created. This is of great importance since provenance information can be used for validation of scientific experiments, auditing of scientific results, and forensic analysis in case of disputes relating to scientific data and discoveries.
ProvLet monitors generation, access, curation, and manipulation of data within 4CeeD’s Clowder system to represent provenance data efficiently via graph structures and visualise results from auditing or forensics queries. ProvLet’s insights are to give attention to the size of provenance logs and have a multi-level visualisation capability to show only need-to-know information (see Video in Figure 2).
A sensory network cyberinfrastructure within cleanrooms allows external sensors such as temperature and humidity sensors to monitor micro-climate around scientific instruments. For scientists, this external sensory data is important for nanofabrication and overall guaranteeing the accuracy of experimentation in cleanrooms. Our SENSELET cyberinfrastructure [4] collects sensory data and forwards them to wireless edge devices and private campus cloud for further analysis, visualisation, and correlation with 4CeeD microscopy data as shown in Figure 3.
SENSELET’s insights stress the correlation of environmental data with the internal instruments’ data that allows for increased accuracy and precision of scientific experiments, and usage of sensory data for maintenance and safety of cleanrooms which is especially important in academic scientific environments.
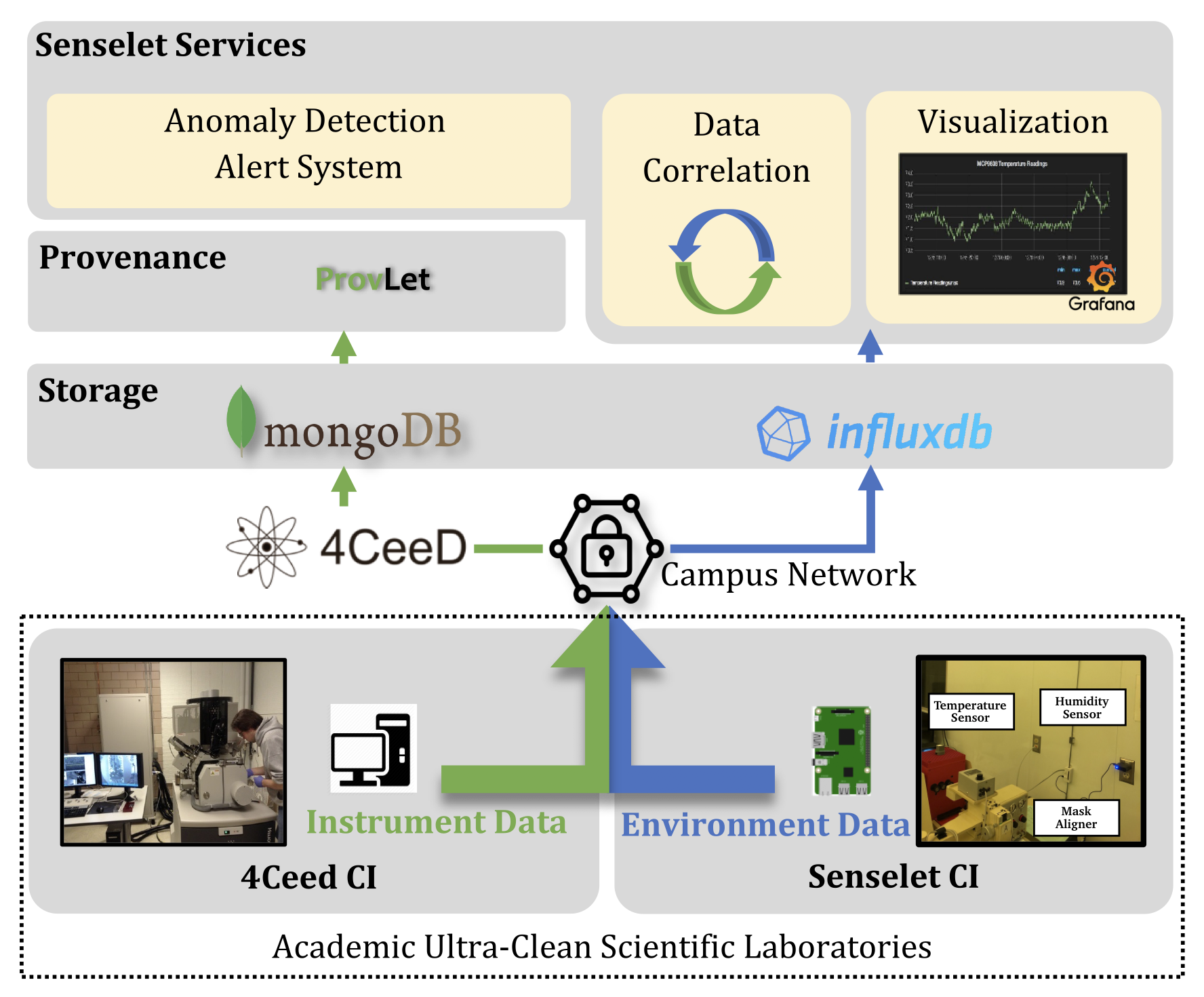
Overall, with increasing challenges in society whether due to climate change, pandemics, or fast technological changes, scientific discoveries are needed to solve the societal challenges, and data-centric cyberinfrastructures in academic ultra-clean scientific laboratories contribute highly to speeding up next generation inventions and innovations that our society needs.
Acknowledgement: This work was supported by NSF grants ACI 1443013, ACI 1827126, ACI 1835834. Any results and opinions are our own and do not represent views of National Science Foundation.
Collaborators: B. Tian, H. Moeini, P. Su, R. Kaufman, S. Konstanty, T. Nicholson, Z. Yang, R. Jain, J. Dallesasse, M. McCollum, G. Pezzarossi, McHenry, T. Smith, P. Braun
References
[1] J.M. Dallesasse, N. El-Zein, N. Holonyak Jr., K.C. Hsieh, “Environmental Degradation of AlxGa1-xAS-GaAS quantum-well heterostructures”, Journal of Applied Physics 68, 2235, August 1990, https://doi.org/10.1063/1.346527
[2] P. Nguyen, S. Konstanty, T. Nicholson, T. O’Brien, A. Schwartz-Duval, T. Spila, K. Nahrstedt, R.H. Campbell, I. Gupta, M. Chan, K. McHenry, and N. Paquin “4CeeD: Real-time Acquisition and Analysis Framework for Materials-related Cyber-Physical Environments”; 17th IEEE/ACM International Symposium on Cluster, Cloud and Grid Computing (CCGrid) 2017, DOI: 10.1109/CCGRID.2017.51
[3] L. Marini, I. Gutierrez-Polo, R. Kooper, S.P. Satheesan, Burnette, J. Lee, T. Nicholson, Y. Zhao, and K. McHenry, “Clowder: Open Source Data Management for Long Tail Data”. ACM Practice and Experience on Advanced Research Computing (PEARC ‘18). DOI: https://doi.org/10.1145/3219104.3219159
[4] K. Nahrstedt, Z. Yang, T. Yu, P. Su, R. Kaufman, I. Shah, Konstanty, M. McCollum, J. Dallesasse, ”Senselet: Distributed Sensing Infrastructure for Improving Process Control and Safety in Academic Cleanroom Environments”, ACM GetMobile 2020, Vol. 24, No. 2, September 2020, doi.org/10.1145/3427384.3427388
Please note: This is a commercial profile
© 2019. This work is licensed under a CC BY 4.0 license.