Tim Simpson, General Manager of Hologic UK & Ireland, discusses how AI, machine learning and prognostics could revolutionise cancer detection and treatment in breast and cervical cancer programmes
The cancer challenge in the UK is deepening – in 2020, 2.9 million people in the UK were living with cancer (1) and another 147,407 people lost their lives to it that year. (2) As the UK cancer registries forecast, unless action is taken, cancer deaths are set to rise by almost 38% by 2030. (3) If we are to address the COVID-19 cancer backlog, as well as focus on reducing lives lost to cancer, then we will need to advance novel approaches in disease prediction and cancer programmes: machine learning combined with prognostics is one such area.
In the pandemic, the government-industry-academia collaboration proved that we are able to come together when the threat is acute.
That level of focused collaboration can now be applied to overcome some persistent challenges to AI’s widespread adoption in clinical settings. In the UK’s 10-Year Cancer Plan (4) from 2022, on AI it says, “AI, digital and data will play an important role in early diagnosis. We will continue the development of unrivalled ‘at-scale’ data infrastructure and make all types of data available, linkable and ‘research-ready’, in a streamlined secure and privacy-protected way.” I am acutely aware that there are serious challenges to overcome including legal and ethical stands, cybersecurity, governance, and personal data protection compliance rules.
However, if they can be conquered, then prognostics, the field of predicting disease progression and outcomes, aided by machine learning, is one of the most exciting areas of medicine. It has the potential to dramatically reduce cancer’s devasting effect on our societies.
Reducing cancer’s devasting effect on our societies
Firstly, it is a matter of collaborating to get data-sharing foundations in place so that machine learning algorithms can then be built to analyse comprehensive global cancer patient datasets. In turn, this will help unlock opportunities in risk stratification and prognostics so that clinicians and researchers can better predict who is at the highest risk of developing certain cancers.
With this knowledge, a more personalised screening pathway could be developed to predict which treatments will suit which cancer patients for better overall outcomes, whether it’s avoiding radical surgeries and invasive treatments or more years spent in better health with family.
Building AI foundations for better cancer prediction
Trust is critical if AI in healthcare can deliver on its potential. It is up to all of us to build trust within the sector, across academia, governments, and industry, so that healthcare data can be accessed ethically, with individuals anonymised and any identifying information removed. AI is only as good as the datasets it is trained on, but we need to treat them with respect and care.
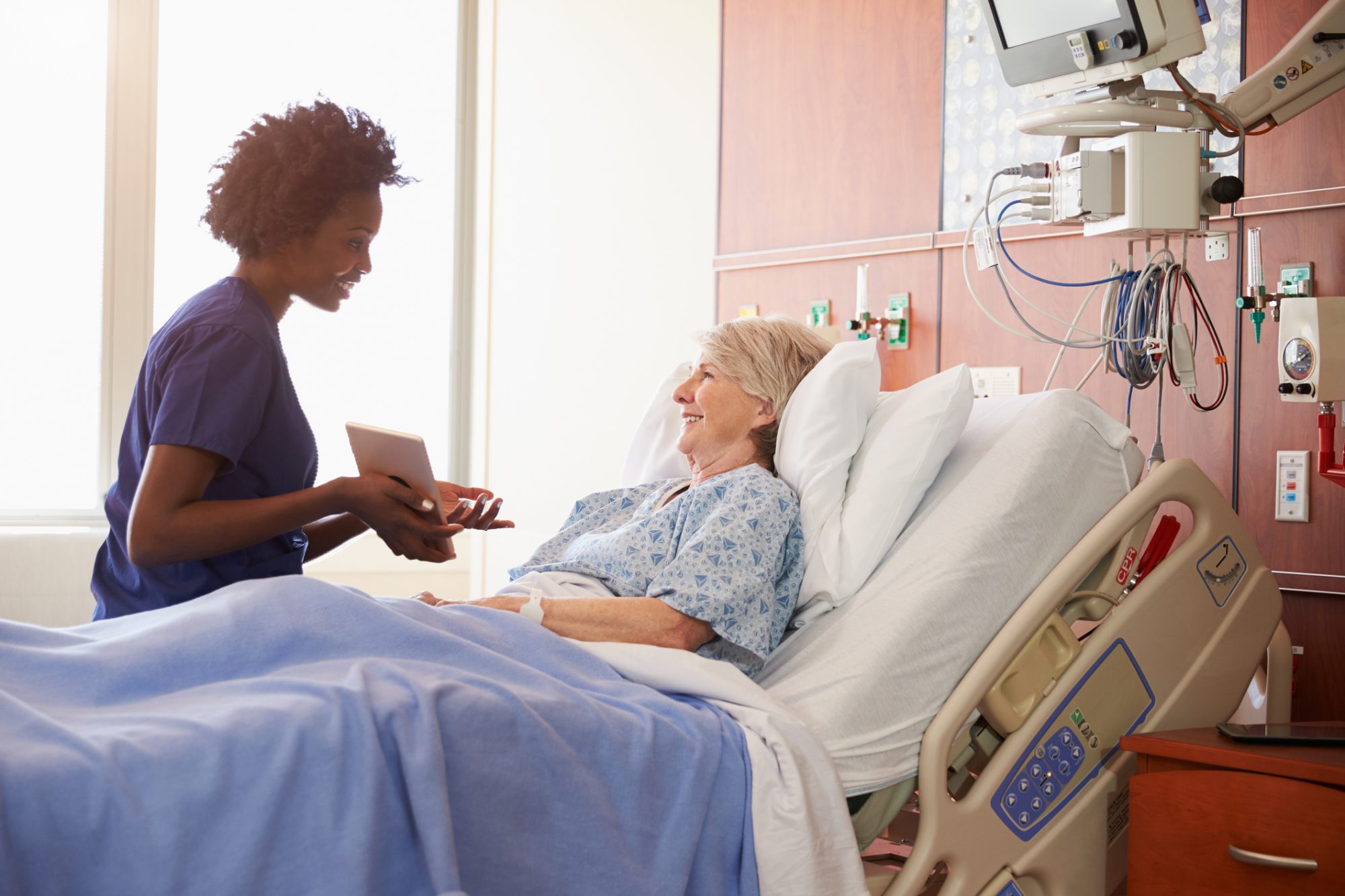
Sharing of electronic health records, compliant with UK data protection rules, is vital to ensure that medical information is shared transparently and efficiently with oncologists, pathologists, radiologists, surgeons, and medical AI developers to enhance the prediction, detection and treatment of cancer. As the UK ‘10-Year Cancer Plan’ notes that an ‘at-scale’ data infrastructure “will provide opportunities to trial AI and machine learning technologies within the NHS – helping to, for example, radically improve our assessment of cancer risk to support more stratified diagnosis and treatment and our ability to identify and diagnose cancers”.
One area where prognostics is already starting to be explored is in breast cancer programmes; there are strong datasets globally where patients have been followed for many years. We can trace back over 10-15 years and see what the mammograms looked like, what the pathology looked like under the microscope, what regimen of chemotherapy was given, other interventions received, and the resulting outcomes. It’s these large datasets that track a high volume of patients with this degree of insight which is necessary to build predictive models.
How prognostics can change the patient journey
By using these large datasets, we can go back to the first mammogram or the first pathology sample from the resection of a tumour and be able to review which patients fared well and which ones did not. We can analyse which ones had a poor or good prognosis, which ones responded to different therapies and responded well, or those patients that had the same treatment but responded poorly.
Then researchers are asking themselves could we have determined that outcome from the very first mammogram or first tissue section from the patient?
That’s the predictive work which is inspiring because of its potential to change the patient journey, and critically the outcomes. It means we’re able to intervene based on what we see in medical images in radiology and pathology and predict, for instance, that a particular patient will respond well to continued oestrogen therapy or different chemotherapy regimens. This kind of innovation in cancer programmes has the potential to radically change patient outcomes.
How do we ensure success in cancer programmes?
Of course, we can talk about the potential of prognostics, which as I’ve outlined will be game-changing to patient outcomes, but we need to make sure we deliver on that potential. To do that we must do three things: ensure the ethical sharing of data, build public trust, and work as a community to drive adoption.
The scientific community is already working with many regulatory bodies to aid the ethical sharing of health data and consider the ethical design of AI platforms for use in medical imaging analysis. We will need to continue these conversations at scale and at speed to ensure AI can become a trusted tool to enable better disease prediction and help us all fight this cancer battle.
References
1. Prevalence by cancer type, nation, sex and year – macmillan.org.uk (no date). Available at: https://www.macmillan.org.uk/dfsmedia/1a6f23537f7f4519bb0cf14c45b2a629/5192-10061/macmillan-2020-cancer-prevalence-figures-and-methodology (Accessed: December 14, 2022).
2. www.ons.gov.uk. (n.d.). Total cancer deaths in the UK in 2019 and 2020 – Office for National Statistics. [online] Available at: https://www.ons.gov.uk/aboutus/transparencyandgovernance/freedomofinfo rmationfoi/totalcancerdeathsintheukin2019and2020
3. Prevalence by cancer type, nation, sex and year. (n.d.). [online] Available at: https://www.macmillan.org.uk/dfsmedia/1a6f23537f7f4519bb0cf14c45b2a629/5192-10061/macmillan-2020-cancer-prevalence-figures-and-methodology [Accessed 28 Oct. 2022].
4. GOV.UK. (n.d.). 10-Year Cancer Plan: Call for Evidence. [online] Available at: https://www.gov.uk/government/consultations/10-year-cancer-plan-call-for-evidence [Accessed 28 Oct. 2022].