Conventional wearable devices mostly rely on motion detection or image classifications to capture users’ activities. What is missing in many existing wearable devices is the decoding of neural signals generated by the human body
Neural signals, such as EEG, ECG, and EMG, offer a rich amount of information on a person’s physiological and psychological activities. Recognition and use of such signals present many new opportunities for applications in medical and daily commercial usage. Recently, artificial intelligence (AI) has been applied to neural signal processing, leading to a new generation of intelligent human-machine interfaces and biomedical devices.
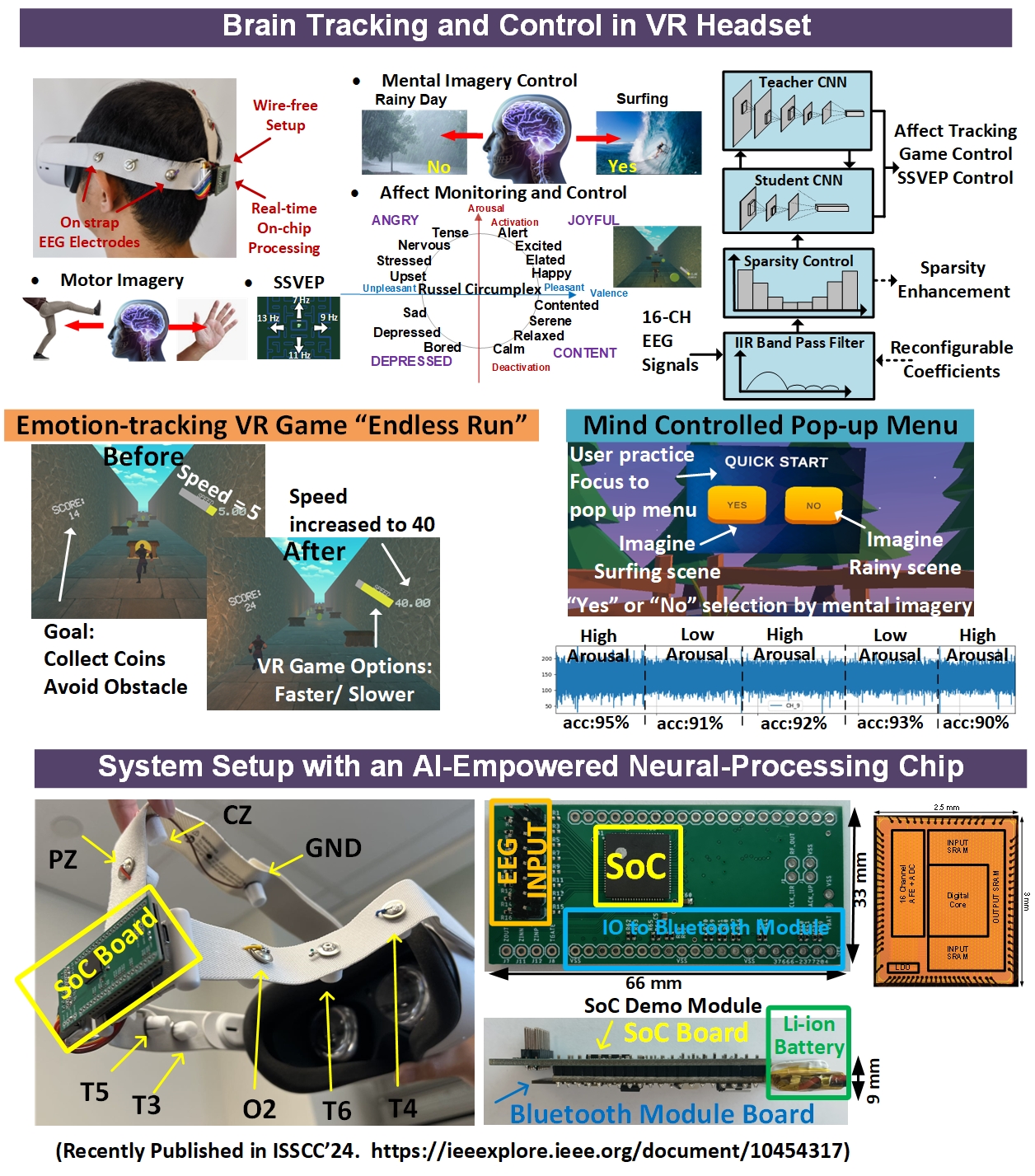
Neural Signals for Physiological Tracking and Emotion Detection
Traditionally, human neural signals such as EEG, ECG, and EMG have found broad applications in human healthcare. For instance, in rehabilitation, patients’ EMG signals are decoded into users’ motion intents, which are further used to control prosthetic arms.
ECG signals have been widely used for cardiac disease diagnosis such as Arrhythmia. EEG signals have been used for detecting anomalies in brain activities such as seizures. For commercial usage, health monitoring, such as heart rate tracking, has been available from existing wearable devices. Interestingly, EEG signals and skin conductance can provide useful information on human emotional states, which can be detected during gaming or video watching to know the users’ mental status. They also have been used for depression studies.
While neural signals are highly useful and informative for tracking human physical and psychological status, their processing and classification are not trivial due to the highly noisy signal representations, human motion artefacts, and highly user-dependent characteristics. This typically requires very sophisticated signal processing methods and feature extraction operations.
Significant progress has recently been made in using artificial intelligence (AI) to perform neural signal processing tasks with high accuracy, low latency, and strong adaptability.
Neural processing empowered by AI for intelligent human-machine interface
Human-machine interface with AI has developed into a large commercial market in recent years. This is mainly driven by the ongoing blooms of Virtual Reality (VR) and Mixed Reality (MR). For instance, Meta has developed an EMG-based wristband assisted by AI technology for human gesture and motion tracking. Similarly, AI has been applied to EEG signals through commercial EEG headcaps for a broad range of “mental imagery” activities, e.g. mind control of joysticks.
Lately, significant interest has been drawn in “mind-to-text” conversion, where EEG signals are collected and translated into natural language through advanced AI models, which usually include a cascade of neural network modules such as CNNs and transformers. Similarly, human emotions are also well-tracked by the classification of EEG and ECG signals using advanced AI models. A deep perception of human neural signals opens up brand new opportunities for machines or computers to understand and communicate with the human “mind” or “body” for intelligent assistance in human daily activities.
Neural processing empowered by AI for biomedical applications
The use of AI for biomedical devices has boomed in recent years. Applications that require neural signal processing are observing high adoption of AI algorithms, which replace many traditional signal processing efforts. For instance, AI algorithms have been applied to EEG for real-time seizure detection.
Similarly, a large body of research has applied CNN for cardiac disease diagnosis with very high accuracy. Due to the complexity of biological neural signals and the commonly large numbers of neural channels used for classification, AI techniques are a natural fit for in-vivo biomedical applications. Recently, Medtronic announced an AI healthcare revolution by investing in AI for real-time medical devices. It is perceivable that AI-enabled neural processing will become the common computing platform for future biomedical devices.
Challenges of supporting AI for intelligent human-machine interface and biomedical devices
While AI models using CNN or the recent transformers are extremely powerful, one of the major challenges is their deployment on small wearable or even implantable electronic devices, which are usually 100x smaller and less powerful than CPU or GPU. A small embedded microprocessor that is commonly used in commercial biomedical devices is not able to process the modern AI models within a targeted timeframe for real-time neural signal processing, e.g. milliseconds for EMG-based gesture classification.
It is well known that such conventional processors using Von-Neumann architecture are very inefficient for AI computing. As a result, there is a recent surge of edge AI devices using dedicated neural network accelerators for processing AI tasks on local small devices. This type of computing device provides 100-1000x better efficiency than existing microprocessors. It is reasonable to expect that the AI accelerator-powered edge AI device will become the hardware choice for the upcoming AI revolution in healthcare.
AI-empowered microelectronic chips in Northwestern University
As one of the leading groups in AI hardware developments for neural processing, Prof. Jie Gu’s team at Northwestern University has developed state-of-the-art AI-enabled neural processor chips that use special neural accelerator architecture to bolster real-time AI computing for neural signal processing.
For instance, a tiny system-on-chip (SoC) solution at a dimension of 2mm by 2mm built from Gu’s lab is able to provide both analog front-end sensing and dedicated CNN/LSTM/MLP AI computing within 1~5ms latency using only 20~50µW, which is 1000x less power than the off the shelf microprocessors on the market. The chips are being used for a variety of biomedical applications such as motion intent detection for prosthetic devices, Arrythmia detection for cardiac diagnosis, etc.
A cognitive chip for supporting VR/MR applications
Recently, a special cognitive chip has been developed for supporting VR/MR applications. The chip is seamlessly integrated into Meta Quest2 VR headset and can classify users’ EEG signals in real-time with electrodes hidden inside the headset without cumbersome wearing as conventional EEG caps.
The integrated VR headset can perform four typical mind control jobs to control the VR scenes, including mental imagery, where the user’s imagination is used to select VR user menu, emotion detection, where the user’s emotions are classified and used to control the game speed, motor imagery where users can use the imagination of limb movements to issue computer command, and finally steady-state visual evoked potential (SSVEP) where special flashing signals on the screen are detected from EEG signals to issue users’ command. All the above point to the great potential AI-based neural processing powered with novel AI accelerator chips bring to our daily lives, workplaces and healthcare.
Future directions and new opportunities
AI has become the “brain” of engineering. By embedding AI into human interface devices, e.g. biomedical devices or wearable devices, we will be able to better understand human desires, activities, emotions and health status. We expect AI-empowered neural processing to become the new computing platform for future human-facing devices in both healthcare and daily assistance.
This will lead to abundant applications on personal mobile devices, human robotics, VR/MR systems, gaming systems, online recommendation systems and biomedical devices. For the next step, enabling online learning and user adoption will be a key feature to be developed to make such devices widely acceptable for general users and patients.
Jie Gu
Associate Professor
Department of Electrical and Computer Engineering
Northwestern University
Brain Tracking and Control in VR Headset Study
2145 Sheridan Road, L473
Evanston, IL