Lester Litchfield, Head of Data Science at Volpara Health, discusses the future of artificial intelligence in breast health
Early breast cancer detection leads to the best breast health outcomes for patients—with five-year relative survival rates in the United States dropping from 99% for “localized”-stage cancer to 29% for “distant”-stage cancer that has metastasized to other areas of a patient’s body.(1) As artificial intelligence (AI) finds its place within the field of medical imaging, its role in early cancer detection is becoming more prominent. But it can also be used to predict cancer before it occurs and help send patients down a path to prevention.
In breast cancer imaging, AI is often applied as decision support when reading mammograms and characterizing suspicious tissue.(2) But AI can also help clinicians predict who may likely develop cancer by offering more intensive screening regimens and other preventative techniques looking into breast health.
AI for automatic, objective, and precise breast density assessment is commercially available and researchers are studying its use as a means to aid in prediction and prevention.(3)
Breast density assessment using AI
Breast density refers to the relative amounts of different kinds of fibrous, glandular, and fatty tissue(4), and is both a strong, independent risk factor for breast cancer(5,6) and a factor impacting the sensitivity of mammography.(7,8)
It is believed that mammography’s sensitivity is affected by the fact that dense tissue can serve to conceal potentially cancerous lesions on an x-ray image.(7,8) Close to 50% of screening-aged patients have dense breasts in the United States, a population that may benefit from supplementary screening.(9)
Traditionally, radiologists visually and subjectively assess a patient’s breast density to place them in one of four categories according to definitions set out in the American College of Radiology’s Breast Imaging Reporting and Data System (BI-RADS®).(10)
Alternatively, AI can be used to automatically assesses the volume of dense tissue present in a patient’s breast on a continuous scale to help ensure a more precise identification and consistent application of screening protocols. Research has shown that radiologists using only visual assessment assign density categories to patients inconsistently, and the variability between how different radiologists categorize density is quite high.(11,12)
The Volpara volumetric breast density (VBD) measure from Volpara® Scorecard™ has shown consistency and reliability in assessing density in multiple independent, peer-reviewed journal articles (13,14,15,16), and was independently reviewed in a 2021 study that showed it had the strongest scientific evidence for efficacy within this sector.(17)
Breast cancer risk prediction using breast density
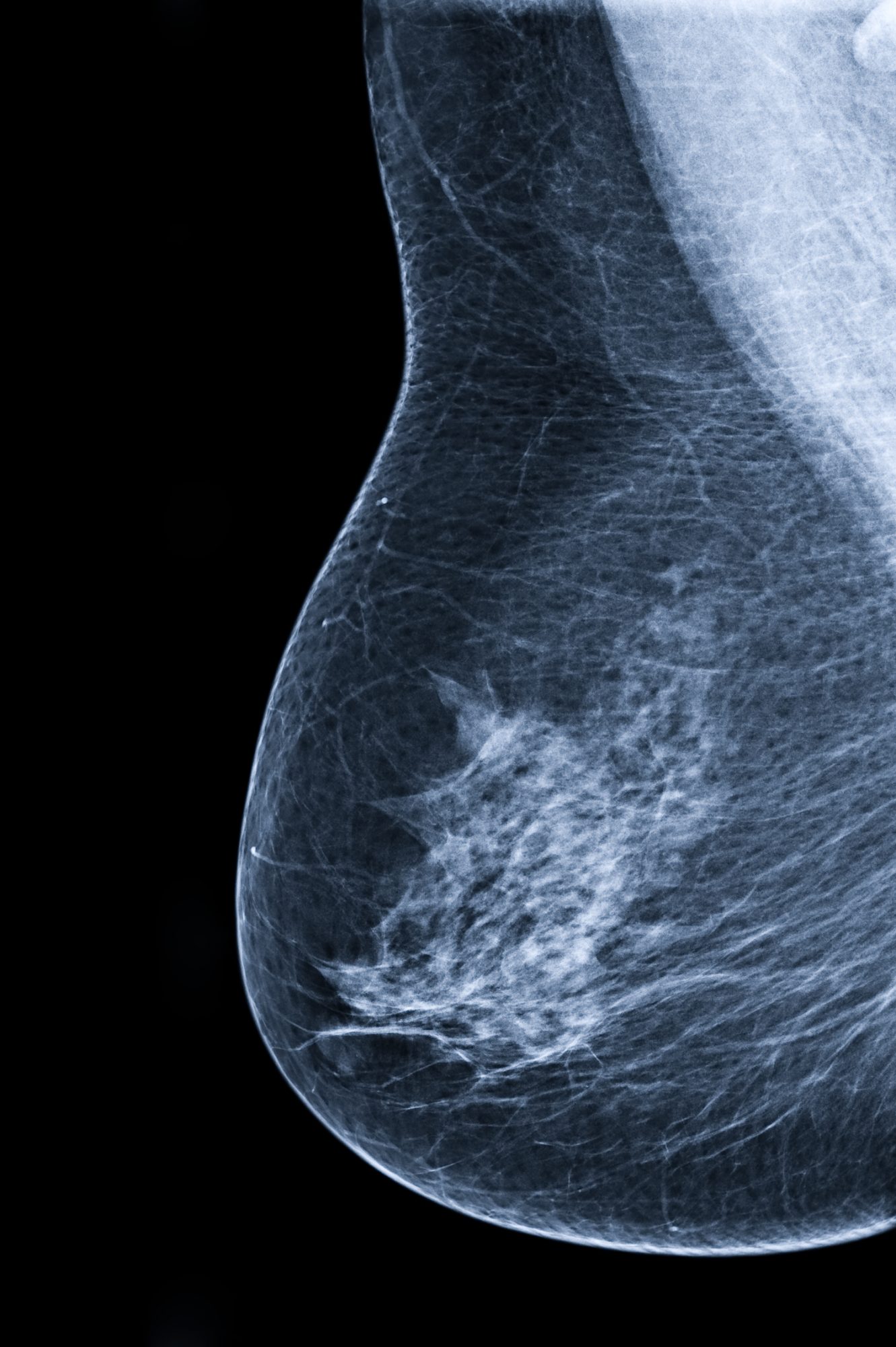
Not only has the use of Volpara VBD demonstrated its benefit in objectively assessing breast density, but it has also proven to be a good mechanism for personalizing breast health care and triaging patients to supplemental screening using alternate screening modalities such as MRI.(18)
Change in medical management toward personalized screening regimens gives patients with dense breasts a higher chance of successfully catching breast cancers earlier and preventing late-stage diagnosis, an d this starts with a risk assessment.
The Tyrer-Cuzick (TC) risk model assesses a patient’s 10-year and lifetime risk of developing breast cancer based on personal and family histories of cancer, as well as other personal risk factors, including their breast density in version 8 of the model. Density inputs to the risk model include the results of the visual assessment (BI-RADS 4th ed., or visual analogue scale) or Volpara VBD (the only vendor density assessment technology integrated with the model).(19)
According to one study in a United States screening setting, using Volpara VBD in the TC risk model resulted in more women being identified as high-risk than through screening alone, making them eligible for additional screening with reimbursement.(19)
Breast density and tumor biology
Aside from general risk assessment, breast density measures have been correlated with tumor characteristics and have been used to strongly predict interval and node-positive breast cancers.
In a study at Elizabeth Wende Breast Care (EWBC) in New York, Volpara VBD had the strongest association with tumor size in the 406 breast cancers studied. Women with category D dense breasts had 3.8 times the risk of being diagnosed with tumor sizes greater than 2 cm compared to women with category A or B dense breasts combined.(20)
A second study at EWBC looked at 318 screen-detected breast cancers and 100 interval cancers and found that the Volpara® Density Grade™ measure (VDG®), calculated from Volpara VBD, had a stronger association with breast tumor characteristics than BI-RADS density scores. For all cancers, VDG was associated with HER2 status and a tumor size greater than or equal to 2 cm, whereas BI-RADS only showed an association with tumor size for screen-detected cancers. Finally, Volpara VBD had stronger associations with tumor characteristics that are associated with poorer outcomes than both VDG and BI-RADS.(21)
A final study from 2021 indicated that Volpara VBD could strongly predict interval and node-positive breast cancers, with women in the highest quartile of dense volume having a 4.5 to 5-fold increased risk for these forms of breast cancer.(22)
Monitoring preventative measures using breast density
Furthermore, breast density has been studied in relation to various chemopreventatives (23) along with some other personal and lifestyle factors, some of which could signal new opportunities for cancer prevention. Three recent studies indicated relationships, or the lack thereof, between breast density and caffeine, cigarettes, and commonly used medications. No significant associations between smoking or the substances studied provided any proof of creating a decrease in breast density.(24,25,26)
AI’s role is constantly evolving. Many researchers, for example, are beginning to develop methods to predict risk and breast health analysis from images(27,28) and to study an individual’s mammography images over successive screening rounds to identify changes over time and whether those changes should indicate the need for a specialized care pathway.(29,30,31) The meaning of these changes still isn’t clear, such as how to elucidate the distinction between early signs of cancer and patterns indicating risk(32) – but AI will be a way to track them and help define them.
AI holds a promising role in the future of breast cancer prediction and prevention, from reaching patients earlier to raising the standard of care. Prevention will only be possible when the effort is taken to assess breast cancer risk at an early age so that appropriate screening regimens and other interventions can be offered and applied to high-risk patients.
References available on request

This work is licensed under Creative Commons Attribution-NonCommercial-NoDerivatives 4.0 International.