Takahira Yamaguchi of Keio University explores how AI smart glasses can act as a smart, simple solution to support maintenance work concerning highway equipment
At Keio University, we develop smart glasses with artificial intelligence (AI) facilities (AI smart glasses) to support the maintenance work concerning highway equipment. This maintenance work requires physical motion alongside various kinds of knowledge, including both formal knowledge from manuals and more subtle, tacit knowledge from skilled workers. Figure 1 shows the system overview of how AI smart glasses function. The combined knowledge & data consists of a workflow, rule base, goal tree to justify rules, domain ontologies (a device ontology and a work process ontology), RDF data, and multimedia (images and videos).
As AI smart glasses support the maintenance work of novice/beginner workers, many questions from the workers during the maintenance work are given to AI smart glasses via voice-control for simple hands-free operation. The questions become 3W1H (what, when, why, how) related to multiple knowledge & data. The answers from AI smart glasses come up on the screen of the smart glasses and are then provided by voice, so it is the case that the voice supports the work.
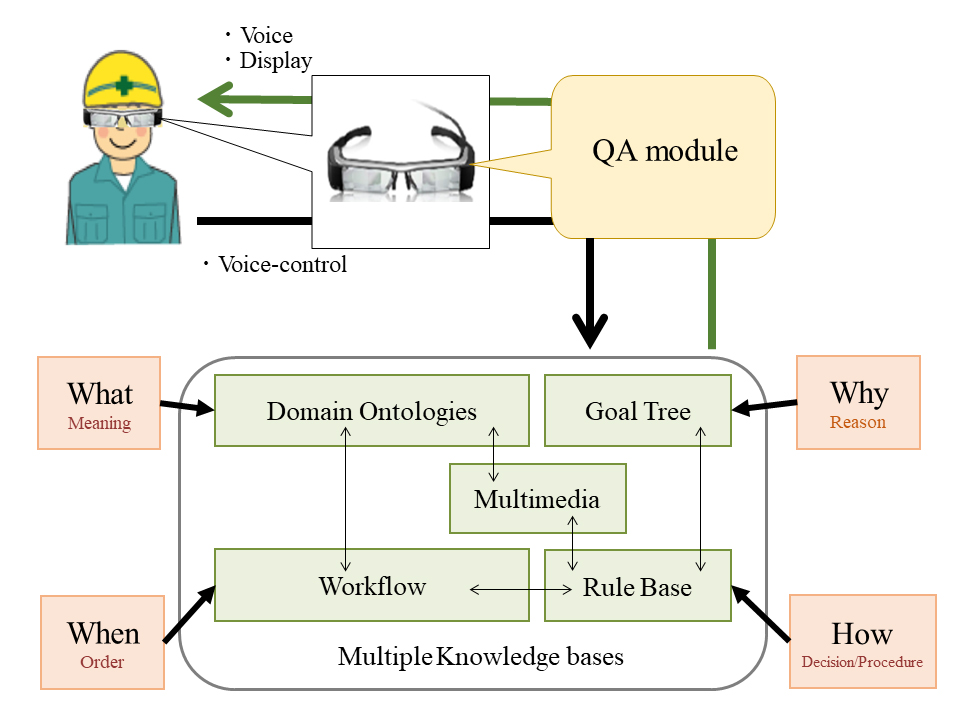
Multiple knowledge & data
Workflow
The workflow shows novice/beginner workers how the maintenance work process goes. It has two levels: macro-level and detail-level. Macro-level workflow presents the whole flow of maintenance work. Detail-level workflow decomposes macro-level work into detailed works. Figure 2 shows the macro-level workflow and decomposed detail-workflow.
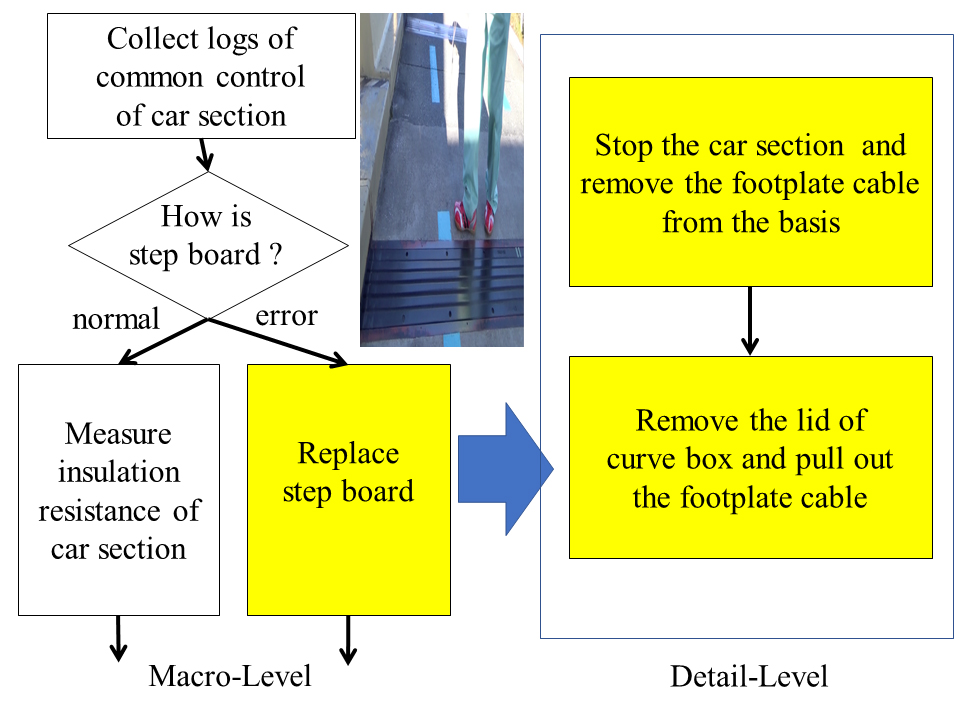
Rule base
IF-THEN rules show how the workers perform each work in a detail-level workflow. IF-part shows some symptom observed by workers and THEN-part shows some action that should be done by them. Novice/beginner workers can do detailed work by themselves using this rule base.
Goal tree
Goal tree explains the rationality of the rules, such as structure and function related to IF-part symptom, efficiency related to THEN-part action, the goal is to perform IF-THEN rules and so on. The explanation facilities with goal tree enable novice/beginner workers to understand why the rule should be taken in performing some work. They understand maintenance work background and can, therefore, adapt existing knowledge to unknown cases.
Domain ontologies and RDF data
Domain ontologies include two kinds of ontologies: a device ontology and a work process ontology. The device ontology defines the structure and function of device components. The work process ontology defines several items related to maintenance work, such as precautions and tools necessary for the work and so on. RDF data corresponds to the instance data of leaf classes of domain ontologies. Figure 3 shows us examples of device ontology and work process ontology and their RDF data.
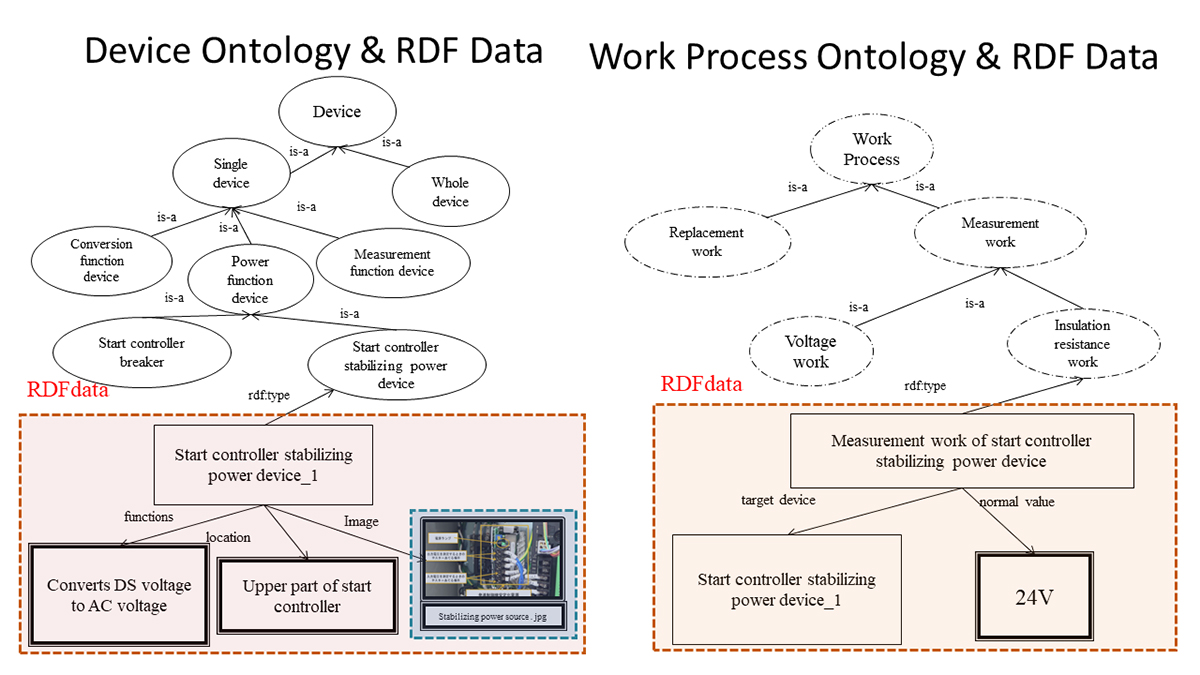
Multimedia
We have taken pictures of device components and edited the images with subtitles. We have also recorded how highly skilled workers perform maintenance work and have added subtitles. The images and videos have been linked to RDF data. When basic instructions are difficult for novice/beginner workers to follow and effectively perform maintenance work, these images and videos become extremely helpful for them to do so.
Question-answering facility with AI smart glasses
The workers put on smart glasses, perform maintenance work, and follow instructions from the workflow and the multimedia that is displayed on the smart glasses. When they have questions about the instructions, they press the earphone button to start the QA (question-answering) facility available with AI smart glasses. So far, the questions haven’t come from the workers themselves, but a list of questions from the QA facility. Answers are displayed on the smart glasses as text by calling the number in the question list. The answers are displayed in the text as the workers perform maintenance work in a loud environment with outside car and wind noise, thus voice communication is often un-effective in this setting.
Case studies and evaluation
As the case studies to evaluate the QA facility, we look at how to inspect the malfunction of an ETC lane on a Japanese highway. Two novices/beginner workers W1 and W2, attend the case studies. Figure 4 and Table 1 shows us how the case studies go and the evaluation results.
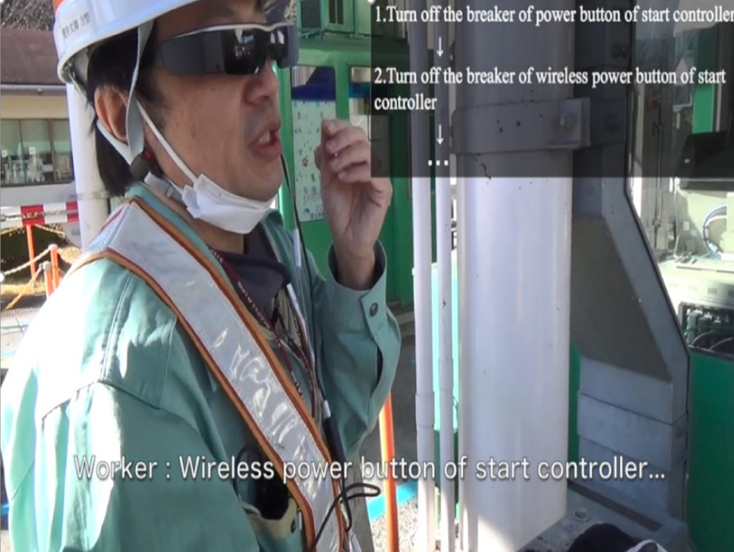
The case studies classify maintenance work into four categories:
- The worker can perform the work and understand its significance without the QA facility.
- The worker can perform the work without the QA facility, but does not understand its significance.
- The worker can perform the work and understand its significance with the QA facility.
- The worker cannot perform the work and understand its significance with the QA facility.
The inspection work becomes more difficult, according to (A) to (D) as follows: (A) means that the worker can perform the inspection and understand its significance just with workflow and multimedia. (B) means that the worker can do it but not understand its significance just with workflow and multimedia. (C) means that the worker needs a QA facility to perform it and understand its significance. (D) means that the worker does nothing even with a QA facility.
As (A) is so easy, and (D) is so hard, the QA facility is not necessarily there. It goes well for the worker to understand the inspection work’s significance in (B) and to perform it and understand its significance in (C). Computing (B+C=8)/(B+C+D=15), QA supports 53% of total work where the worker needs some support facility in (B) to (D). The analysis of (D) means the following is difficult to tell novice/beginner works: where is located device component? How to explain perceptual information?
Conclusion
As the maintenance works include the actions with refined skills, we should integrate multiple knowledges and movies in details. As the number of workers is declining, who support social infrastructure related to transportation and electric power, AI smart glasses will be even more important in the future.
Please note: This is a commercial profile