Here, Arianna Agosto, Paolo Giudici, Paolo Pagnottoni and Anca Mirela Toma discuss how a research-driven European environment can create sustainable digital finance for Fintech risk management
Financial Technologies (FinTech) arise when new technologies, such as big data analytics, artificial intelligence (AI) and blockchain are applied to develop new financial services. FinTech brings many opportunities for business and consumers. But which are the risks related to the new financial technologies? And what are the Regulators doing to understand whether Fintech is reliable and trustworthy?
In 2018, the European Commission decided to fund a research project aimed at creating an open-source risk management environment for fintech applications, such as new lending platforms, blockchain payments and robot advisors powered by AI techniques. The FinTech project received €2.5 million funding within the Horizon 2020 Research and Innovation programme. It involves 24 partners (21 universities and 3 fintech companies, coordinated by the University of Pavia Fintech Laboratory) and financial regulators from all European countries. The project activities started in January 2019 and will continue until December 2020. Academics and professionals are working together to build explainable AI data-driven models that can measure and, therefore, manage the new risks emerging from digitally innovated banking and finance.
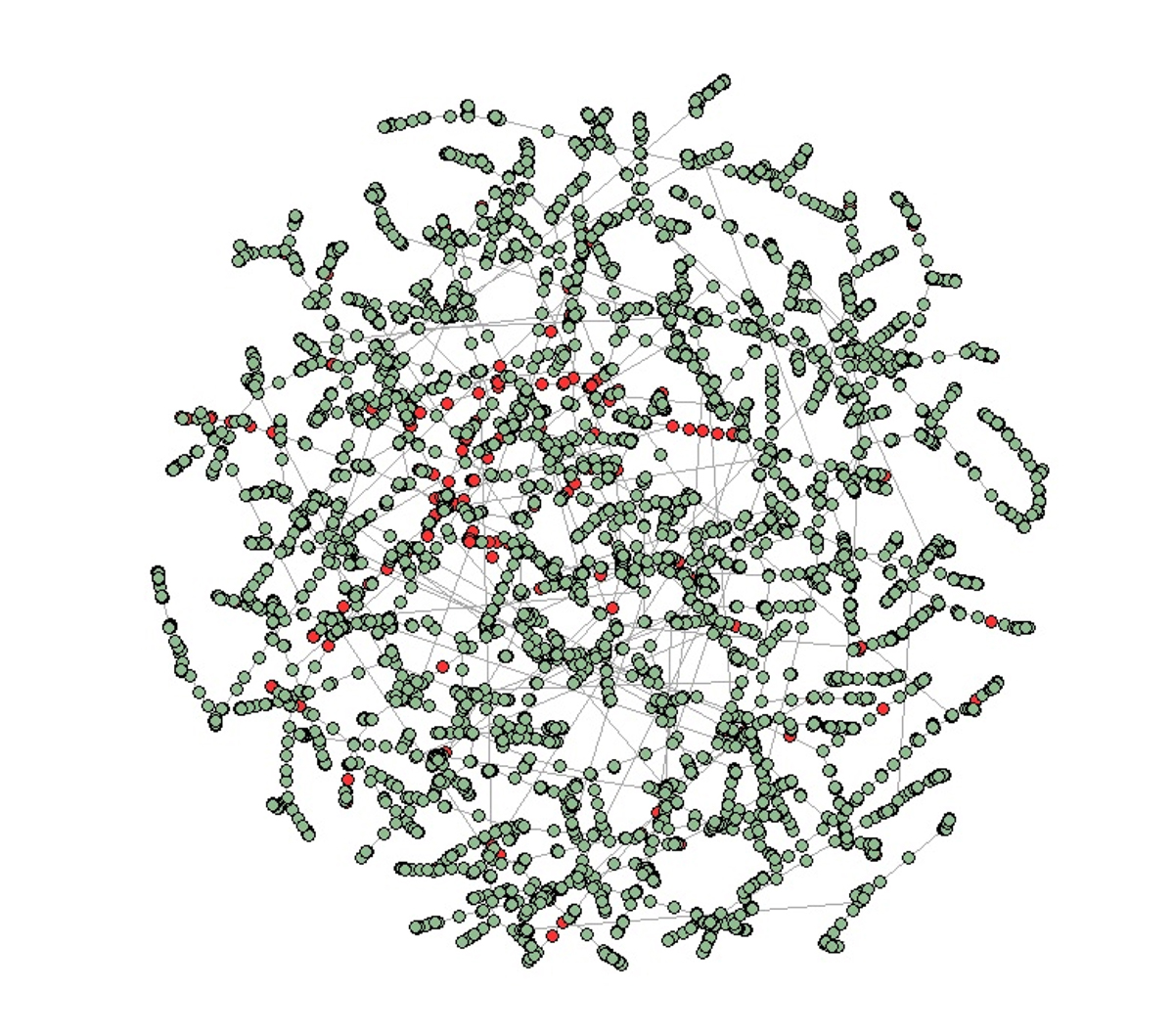
Based on the analysed risks, the project research can be divided into three distinct, albeit related, parts: Credit risk management in peer-to-peer lending (Part 1), Market risk management in robot advisory asset management (Part 2), Operational and cyber risk management in blockchain payments (Part 3).
For each part, the project develops and shares a collection of risk management use cases with financial national regulators in all European countries. At the European level, besides the European Commission, the European Banking Authority (EBA), European Securities and Markets Authority (ESMA), European Insurance and Occupational Pensions Authority (EIOPA) and the European Central Bank (ECB) are involved: together with international authorities such as the Financial Stability Board (FSB), International Monetary Fund (IMF), Bank for International Settlements (BIS), Organisation for Economic Co-operation and Development (OECD), they are asked to give their feedback on the project use cases during six “research workshops”, which take place at the beginning and at the conclusion of each project part. The research workshops are open to anybody, upon registration, and put together the views of academics, regulators and financial companies, including both traditional banks and fintechs.
For each of the three project areas, before the final workshop, the use cases are presented to the national supervisors (central banks and market authorities) during the “SupTech” events, where the first feedback exchange takes place.
Fintech companies and banks can join dedicated events, called “RegTech”, where coding sessions allow professionals to get in touch with the developed cases in a practical way. During and after the RegTech events, banks’ and fintechs’ representatives can test the models operationally, downloading codes, data and papers related to each use case from the project platform. Besides the workshops, all the project stakeholders can access the platform at any time, upon registration.
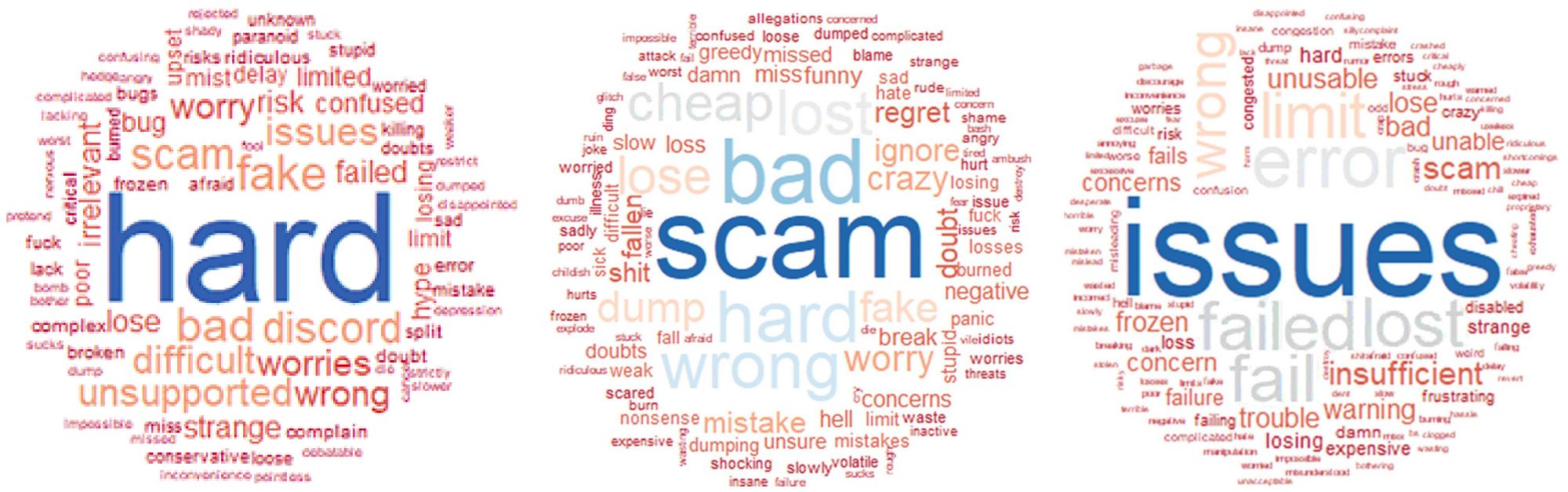
From a technical viewpoint, the models developed for credit risk in peer-to-peer lending are based on the use of network theory to measure connectivity between economic agents. Indeed, in the new lending platforms private individuals are able to directly provide small and, in most cases, unsecured loans to private borrowers and/or small and medium enterprises (SMEs). This increases contagion risk without the platform being responsible for credit risk assessment, differently from traditional banks.
A network model based on balance sheet similarities between the borrowing companies is applied in the first use case developed by the project (Giudici et al., 2019), while in the second use case Ahelegbey et al. (2019) improve credit scoring models by clustering SMEs based on latent risk factors, deduced from financial ratios. Both studies use real data coming from peer-to-peer lending platforms, which are also used in a third use case (Agosto et al., 2019), where a network is built upon trade flows between the companies joining the platform.
Information about the interconnectedness generated by fintech platforms is also leveraged in Part 2 of the project research, which is currently ongoing with new use cases being presented to the national supervisors. In particular, Pagnottoni and Polinesi (2019) show that the correlation between financial assets – increasing in crisis periods – is central in assessing contagion risks that can sensibly affect market risk arising from robot advisory asset management services.
Protection of investors is also crucial in innovative payments such as Initial Coin Offering (ICO), where funds are raised through the use of blockchain-based digital currencies (cryptocurrencies). Unfortunately, many ICOs are performed by non-reputable entities, which disappear after raising large amounts of money. Predictive textual analytics methods, allowing to discriminate between successful and fraudulent activities, is one of the techniques applied in the challenging Part 3 of the project, related to the measurement of operational risks in blockchain payments, as the seminal work of Cerchiello and Toma (2019).
Authors: Arianna Agosto, Paolo Giudici, Paolo Pagnottoni and Anca Mirela Toma
Disclaimer
All material presented here reflects only the authors’ view. The European Commission is not responsible for any use that may be made of the information it contains.
References
Agosto, A., Giudici, P. and Leach, T. (2019). Spatial Regression Models to Improve P2P Credit Risk Management. Frontiers in Artificial Intelligence, 2:6.
Ahelegbey, D., Giudici, P. and Hadji-Misheva, B. (2019). Factorial Networks Models to improve P2P Credit Risk Management. Frontiers in Artificial Intelligence, 2:8.
Cerchiello, P. and Toma, A. (2019). Protecting investors: Detecting fraud in ICO through textual analytics. Under revision.
Giudici, P., Hadji-Misheva, B. and Spelta, A. (2019). Network Based Scoring Models to Improve Credit Risk Management in Peer to Peer Lending Platforms. Frontiers in Artificial Intelligence, 2:3.
Pagnottoni, P. and Polinesi, G. (2019). Network models to reduce robot advisory market risk. Submitted.
Please note: This is a commercial profile