Craig Rhodes, EMEA Industry Lead, AI for Healthcare and Life Sciences, NVIDIA, argues that just four tools could enable the future of AI in healthcare
From AI-generated synthetic brain images used for studying brain diseases like Alzheimer’s, to identifying and tracing cancer tumors to target with radiation therapy, AI in healthcare has been used by professionals across the field to help with the toughest challenges.
But as AI moves out of research and gains traction in clinical care and treatment, there are a number of critical barriers remaining that will determine the success or failure of AI in healthcare and life sciences.
These are challenges that have dogged the world before AI and will continue to cause roadblocks that need to be addressed. To overcome these, we must design solutions that ensure data sovereignty, ethics, and patient privacy are considered and respected at each step of the way while adopting new techniques such as synthetic data and federated learning to share data in the right ways.
Adoption of AI ethics & governance policies
Europe’s landmark GDPR regulations are templates for healthcare AI, but governments will need to go much further.
AI systems should not be a black box and patients should be educated on how their data is being used in the process. There is also the issue of fragmentation, between where the data actually lives and where it might be used.
How the AI will be used and ensuring the data it was trained on do reflect the diversity of the population will need to be factored in for each algorithm use case. Startups must make focusing on ethics a key initiative because this work takes some time. This work should be done in parallel with the development of algorithms to ensure they both stay top of mind.
Ensuring those algorithms have been trained and annotated accurately is paramount, in order to avoid the wrong conclusion and to ensure the correct diagnosis is made.
It’s similar to the work being done for autonomous vehicles, ensuring training data is correct to keep the roads safe.
Curation of annotation
The availability of well-annotated data, by trained experts, is another hurdle.
Programs like the London Medical Imaging Center and AI Centre for Value Based Healthcare, PathLAKE, and the Industrial Centre of Artificial Intelligence Research in Digital Diagnostics (iCAIRD), are seeing the volumes of data skyrocket exponentially, going from just a handful of images to a million in the span of a year.
This work is now moving from a great technical engineering exercise to the laborious work to rapidly label clinical data while ensuring it is done accurately.
Just as an expert consultant and pathologist would diagnose a patient with cancer, we need to ensure the same care is carried out when annotating a pathology image that will go into an algorithm at the point of care for cancer treatment.
As data volumes are increasing, we need to be able to keep up with the data curation and annotation to ensure that it is valuable for AI. We are now seeing techniques being developed for semi-automated annotation, providing a helping hand for this task.
Data access & federated learning
In addition to leveraging AI for data ingestion and analysis, federated learning techniques will enable improved data sharing across departments, jurisdictions, and companies because of its ability to maintain compliance with data sovereignty and privacy regulations.
Federated Learning is a privacy-preserving technique that brings the AI model to the local data, trains the model in a distributed fashion, and aggregates all the learnings along the way. In this way, no data is exchanged or left the healthcare institution. The only exchange occurring is model gradients.
Programs like the AI Center for Value Based Healthcare are utilising federated learning to build more robust AI models across hospitals and trusts.
Similar public and private partnerships can build on an open-source platform to ensure data remains private and does not leave the institution.
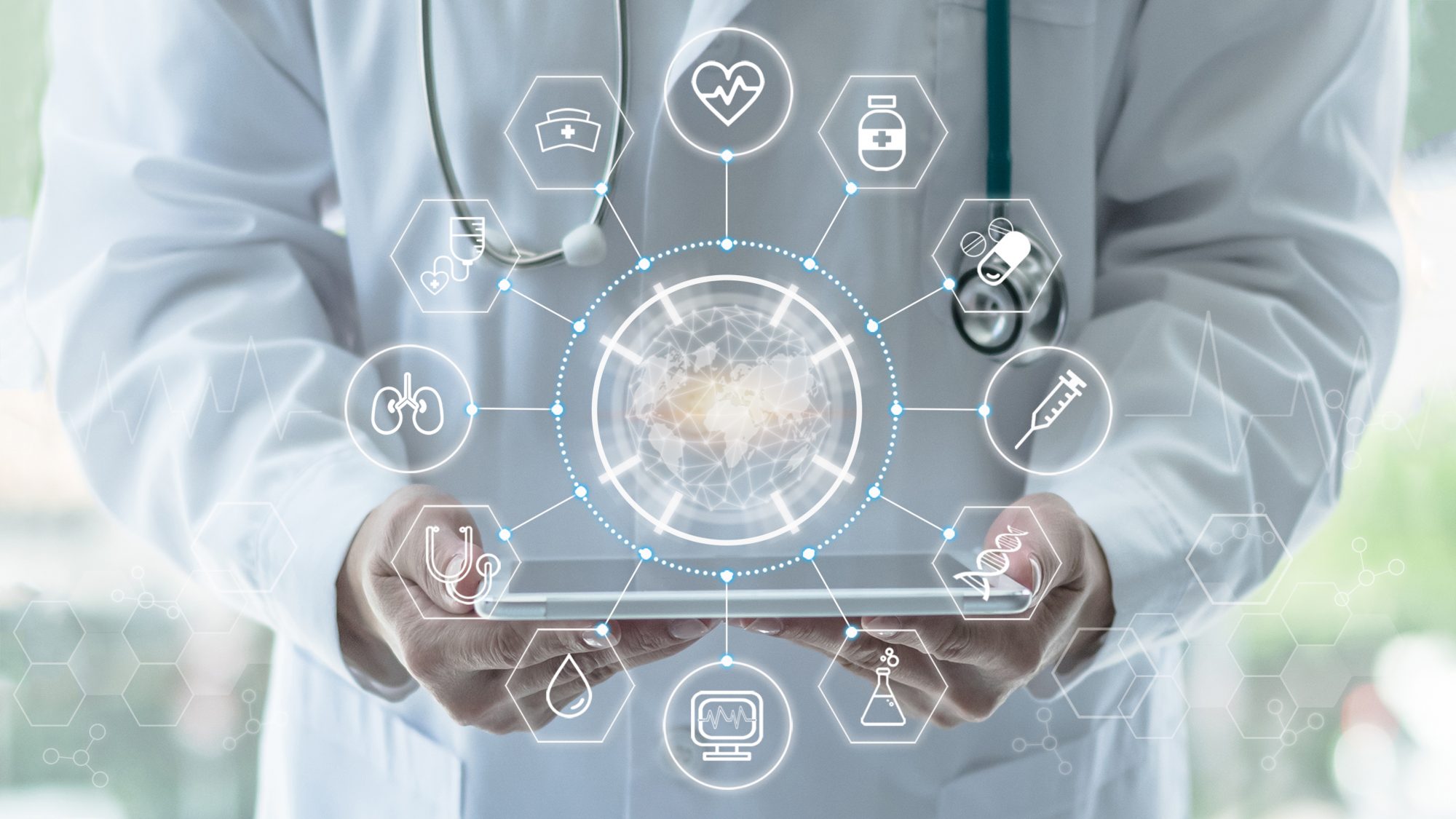
Employ the power of synthetic data
Synthetic data offers the opportunity for researchers to create tools, models and tasks without risks or privacy concerns.
The data maintain the characteristics of health records it was trained on, but these AI-generated records could be used to supplement and balance in datasets to represent the patient population better and help rule out bias.
For example, research institutions could use synthetic data to create health records of digital diabetes patients that have features similar to a real population.
King’s College London is working to generate synthetic brain images to better understand the progress of brain diseases like Alzheimer’s with the goal of improving diagnosis and treatment.
Since synthetic data simulates real data, without being associated with patients, this can be readily shared across research institutions without raising privacy concerns.
Transform the potential of medical AI into reality
To drive a wide adoption of AI in healthcare, collaboration and coordination across governments, industry, and technology is needed to address the challenges with data, governance and ethics.
The need for a medical ecosystem that supports the use of AI is an important one. Without it, the exciting medical research in AI happening today will not continue to be enabled.
These tools will start to accelerate AI past the challenges, transforming its momentum into a steadfast force for innovation in the industry.