Dr Jeanine Houwing-Duistermaat (statistics) and Dr Gastone Castellani (biophysics) from the University of Bologna, Italy, organised innovative interdisciplinary training in multi-omics research within the IMforFUTURE project, which focused on communication between wet and dry lab
The next goal after the IMforFUTURE project is training on use of historical data and current knowledge in omics research.
Biomarkers
Evidence-based decision making is essential for effective healthcare. Its philosophical origins extend back to mid-19th century Paris and earlier, and it involves the conscientious, explicit and judicious use of current best evidence in making decisions about the care of individual patients. The practice of evidence-based medicine means integrating individual clinical expertise with the best available external clinical evidence from systematic research. However, systematic research when there is a tsunami of omics data is not simple.
Biomarkers play an important role in these decision-making processes. They are used for diagnosis, monitoring disease progress, and choosing the best treatment. Omics research is promising to deliver cheap and accurate biomarkers. It includes genome-wide DNA markers reflecting the genetic code, transcriptomics that quantifies expression of genes, proteomics measuring the abundance of proteins, and glycomics that studies sugar molecules surrounding and modifying proteins in your body. For example, glycomics resulted in the omics-based biomarker GlycanAge®, which can be used to assess how old someone is in biological age, rather than chronological age. This can be useful if you are elderly, and a decision needs to be made about whether surgery is a good idea or not.
Reuse of omics data
Omics research has produced many datasets and many efforts have been made to make these data and the results of their analyses ‘findable’. In practice, this means that much omics data is freely available and can be downloaded and re-used. Bioinformaticians have also realised that these historical data and results are not straightforward to use for an individual researcher. They have developed algorithms to summarise the available information.
For example, algorithms exist that count the number of times two genes are mentioned together in a scientific paper. Scores have been developed which combine these counts with results from experiments and models for biological systems. The higher the score, the more likely it is that the two genes interact and are involved in the same biological processes. Although these scores provide a summary of current knowledge, it is still not clear how to use them in a systematic way to facilitate decision making.
Statistical consulting
Jeanine: “As a PhD student in Medical Statistics at the end of the previous century, I performed statistical analyses for clinicians. Typically, I had to estimate the strength of the relationship between a marker and an outcome variable. At that time, I only needed information about the design of the study and the targeted population for which the researcher wanted to infer the relationship. This information was needed to formulate the assumptions under which my identified relationship between the marker and outcome would hold. A simple example is when the cases are mostly males while the controls are mostly females, the results are only interpretable when there is no difference in disease incidence and distribution of the marker between males and females.”
Interdisciplinary research
Nowadays, the datasets and the research questions are more complex. We analyse multiple omics datasets instead of one marker. Each omics dataset has a huge number of features. Interdisciplinary approaches are needed for efficient and appropriate analyses of these data. IMforFUTURE was a training network in biochemistry, epidemiology and statistics for early career researchers and focused on collaborations between wet and dry lab. For a data scientist, it is crucial to know: What type of data is in the database? How was the quality of a single measurement determined? On the other hand, the chemist needs to know that their handling of samples may affect downstream analysis of the data. For example, if a lab technician decides to measure a few samples twice, this information is relevant for the data scientist in choosing the right model. Ignoring this information may lead to false findings.
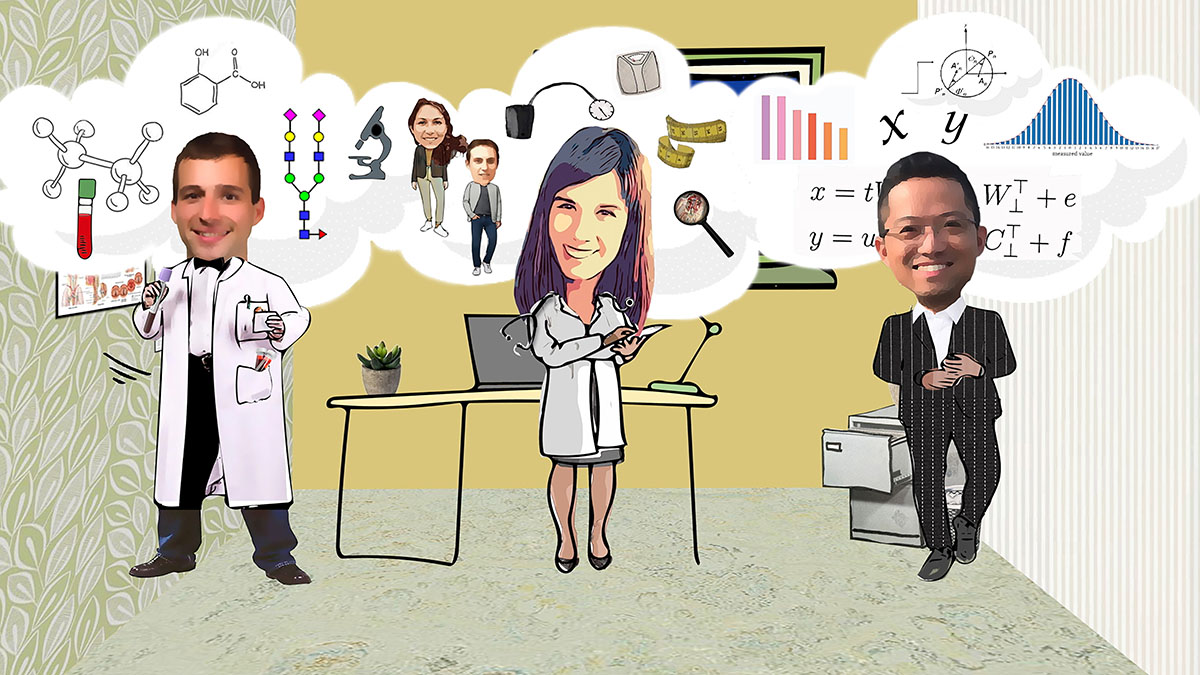
Gastone comments: “Biomedical researchers are not satisfied with the results of omics data analyses. They ask for an interpretation, for actionable information. They want to rank results for further research by specific experiments. Pressing requests are optimal treatment planning, drug repurposing and disease trajectories predictions. This requires input from biophysicists and biochemists.”
The first step is to use historical data and bioinformatic scores to augment the omics data. However, there are no guidelines on how to use these data and scores. Which database to use? Which information is to be used in the score? How to address the overrepresentation of cancer-related datasets? Thus, although the availability of data should enhance the reuse of research, the variety in bioinformatic scores and historical data sets and lack of guidelines, leads to arbitrary and not well-reported choices in omics research.
Next-generation of omics researchers
Efficient, accurate and optimal biomarker development requires communication among biochemists, clinicians and data scientists, including statisticians, biophysicists, computer scientists, bioinformaticians. Bringing PhD students and early career researchers from different disciplines to work together on different aspects of biomarker research is a key to understanding how single choices along the research path affect accuracy and efficiency in omics research. By networking and interdisciplinary training, the new generation of scientists will develop the cross-discipline understanding necessary to contribute to evidence-based decision making in healthcare.
Innovative training in methods for future data (IMforFUTURE) has received funding from the European Union’s HORIZON 2020 Research programme under the Grant Agreement no. 721815.
Please note: This is a commercial profile
© 2019. This work is licensed under CC-BY-NC-ND.