Here, we find out about the research of Christian Nansen from UC Davis, Department of Entomology and Nematology in California concerning spectral repeatability – that is cracking the code to unleash optical sensing in 21st-century precision crop management
Christian Nansen at the University of California Davis and collaborators are developing optical correction models that will greatly enhance the accuracy and sensitivity of optical-based technologies in the precision management of field and greenhouse crops.
Remote sensing, machine vision, and optical sensing are just some of the terms used to describe the use of spectral signals in the detection and classification of objects, such as crop plants. In agricultural crop management, unique and detectable “optical features” in leaf reflectance are identified and used to differentiate “healthy” versus “stressed” field or greenhouse crop plants.
Such unique optical features may represent subtle but highly complex physiological responses to abiotic (i.e. drought, frost, salinity) or biotic (i.e. diseases and insect pests) stressors [1]. Across agricultural sectors, labour shortages and, therefore, needs for automation, growing concerns about environmental consequences of excessive use and reliance on pesticides, and internet and technological advancements are some of the key drivers behind considerable research efforts and private sector investments into the widespread use of optical sensing technologies in precision crop management.
Advanced optical sensing
Similar to image-based diagnostics of diseases/stress factors in humans, early detection and accurate diagnosis of emerging crop pest outbreaks can enable containment of outbreaks with precision-delivery of pesticides and/or precision-releases of biological control agents [1-3]. Such detection of stressed crop plants often relies on optical leaf reflectance features, which are highly subtle and not be visible to the human eye.
Furthermore, healthy and stressed crop classes are not discrete but continuous along a range of stress levels with somewhat arbitrary thresholds to divide crop plants into, for instance, low, medium and high-stress levels. To complicate the classification challenge further, a plant stress response may be a composite to one or several stressors, and younger versus older crop plants vary in terms of leaf reflectance. Hyperspectral imaging data have a high spectral resolution, which enables the detection and diagnosis of subtle crop stress responses. Hyperspectral imaging devices can be mounted on drones, robotic rail systems, or in other ways moved above crop canopies in fields or greenhouses.
In addition, optical sensing data can be acquired with artificial light or with the sun as a light source (Figure 1).
Low spectral repeatability
Probably the single biggest challenge to widespread adoption of hyperspectral optical sensing in precision crop management is directly linked to the inconsistency of sunlight over space and time, as it leads to low “spectral repeatability”. Spectral repeatability can be illustrated based on the minimum-maximum range of reflectance data from objects that do not change over time and are imaged multiple times under similar conditions.
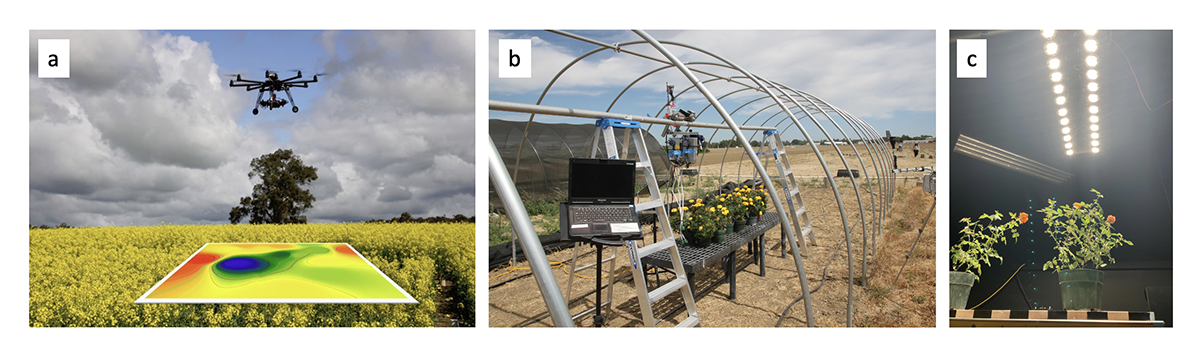
Cracking the code…
Subtle changes in atmospheric composition, cloud cover, and sun angle are partially responsible for inconsistencies in sunlight intensity and radiometric composition, and this complex of spectral and meteorological factors is largely responsible for the low spectral repeatability (wide minimum-maximum range) shown in Figures 2b and 2c. Thus, concurrently with the acquisition of optical sensing data, sensors can be deployed to collect data on sunlight intensity and radiometric composition, cloud cover and sun angle, and these auxiliary data are to calibrate optical sensing. Figures 2d and 2e show that the advanced calibration of reflectance profiles from cones markedly increased the spectral repeatability (reduced minimum-maximum range). Thus, when the same advanced calibration models are deployed to optical sensing data from crop leaves, then the likelihood of accurately and reliably detecting and diagnosing emerging crop stressors have increased markedly.
Tremendous research progress is being made in the development of powerful computers and classification functions, and such innovations will in many and important ways revolutionise aspects of agricultural crop productions. But to fully unleash the potential of optical sensing in future precision crop management, technologies should either rely on artificial and, therefore, more consistent lighting [4] or advanced calibration models will be needed to account for the inconsistency of sunlight over space and time and, therefore, to increase the spectral repeatability.
Optical-based classification algorithms are only as good as the training data being used to develop them, and this is particularly relevant to highly advanced machine learning and deep learning functions, in which model over-fitting may be a concern. In academia, multi-disciplinary courses and both fundamental and applied research are needed to develop academic capacity, recruit students, and fully explore the potential of optical sensing as a frontier in precision crop management.
References
- Nansen C, Murdock M, Purington R, Marshall S: Early infestations by arthropod pests induce unique changes in plant compositional traits and leaf reflectance. Pest Management Science 2021.
- Nansen C, Elliott N: Remote sensing and reflectance profiling in entomology. Annual Review of Entomology 2016, 61:139-158.
- Teske A, Chen G, Nansen C, Kong Z: Optimised dispensing of predatory mites by multirotor UAVs in wind: A distribution pattern modelling approach for precision pest management. Biosystems Engineering 2019, 187.
- Nguyen HDD, Pan V, Pham C, Valdez R, Doan K, Nansen C: Night-based hyperspectral imaging to study association of horticultural crop leaf reflectance and nutrient status. Computers and Electronics in Agriculture 2020, 173:105458.
*Please note: This is a commercial profile