Vittorio Cristini1,2, Eugene J. Koay3, and Zhihui Wang1,2
1Department of NanoMedicine and Biomedical Engineering, University of Texas Medical School at Houston, Houston, TX 77054, USA
2Brown Foundation Institute of Molecular Medicine, University of Texas Medical School at Houston, Houston, TX 77030, USA
3Department of Radiation Oncology, University of Texas MD Anderson Cancer Center, Houston, TX 77030, USA
Cancer is a complex and heterogeneous disease. Its growth, invasion, metastasis, response to treatment, and therapeutic resistance all depend on multiple genetic and environmental cues. Currently, the mainstays of treatment for most forms of cancer are chemotherapy, radiation, and surgery. Unfortunately, many patients fail these conventional treatments, and it is difficult for physicians to predict a treatment outcome with certainty. Despite advances in science and technology as well as significant investments in basic and clinical research, cancer still remains a major cause of death in the United States and globally. New ways of thinking about this disease and treating it are desperately needed. We view cancer as a combination of physical and biological problems, rather than a purely biological one, and this approach may help improve patient outcomes.
Fundamental physical processes in tumors, such as the movement of nutrients, delivery of drug molecules, and exchange of mechanical forces between cancer cells and the surrounding tissue, depend on many biological processes, including the growth of blood vessels, formation of fibrous collagen matrix, and cancer cell proliferation. These biological processes exert physical forces on the cancer cells and ultimately influence the growth patterns of the tumor and its response to treatment. By developing mathematical models to describe these growth patterns and tumor responses to therapies, we recast the biological problem into a bioengineering one. Our approach is clinically relevant because we use quantitative measurements from standard-of-care diagnostics to render our mathematical predictions. Hence, physicians at the forefront of treating cancer patients will have quantitative tools to assist them in designing therapeutic regimens for an individual patient, beyond current subjective and heuristic assessments. In fact, the idea of using mathematics and physics to optimize processes is highly successful in many other industries, including finance, oil and gas, computers, and the automotive industry; so we believe similar quantitative and objective approaches can benefit oncology.
For example, we published promising results towards development of a predictive tool of cancer progression and therapeutic outcome. We demonstrated accurate predictions of tumor kill from chemotherapy in patients with colorectal cancer that metastasized to the liver and in glioblastoma (Pascal et al., Proc Natl Acad Sci U S A 2013; PMC3761643). This model uses measurements of the physical properties of a tumor, including the volume of the tumor that is occupied by blood vessels, the typical size of the blood vessels, and the typical distance among blood vessels. These measurements can be made directly from the tissue or indirectly from diagnostic imaging. With these relatively simple measurements, we can predict how much of a cancer can be killed by drugs with the model (see Fig. 1). We then validated and confirmed the model on almost 200 patients with pancreatic cancer (Koay et al., J Clin Invest 2014; PMC3973100). This paper highlighted the substantial variability of drug delivery between patients and within tumors. Importantly, the variable drug delivery was described by physical transport phenomena that could be measured from standard of care CT scans.
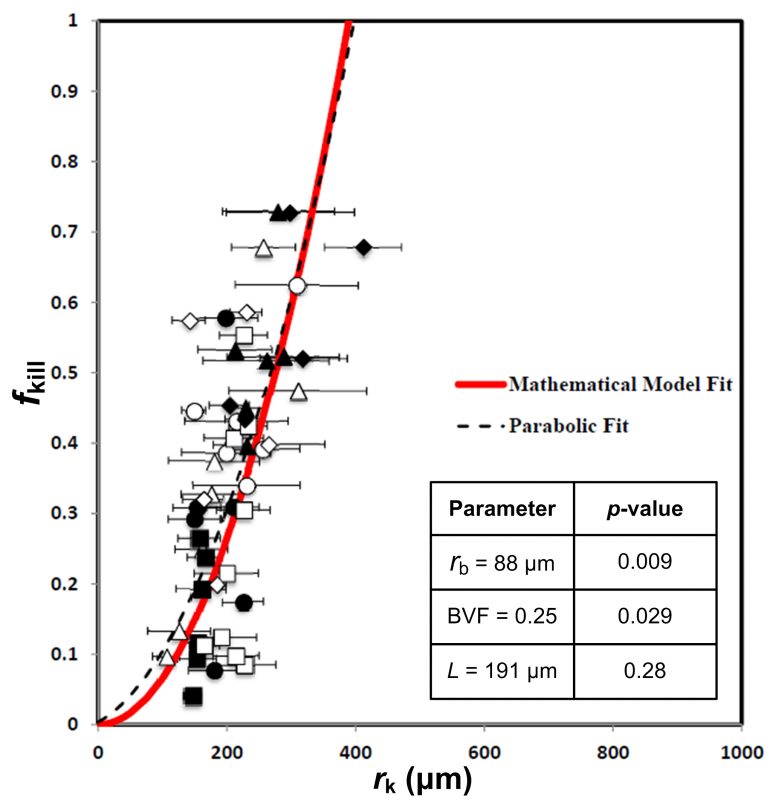
These models are a major step forward for mathematical oncology, but more research is needed to translate these models to patients in the clinic. In particular, we have scientific and practical problems to overcome. For example, we need to understand these physical phenomena in tumors in the contexts of emerging therapeutic strategies like targeted drugs, immunotherapy, metabolic therapy, and nano-particles. In collaboration with an interdisciplinary network of colleagues from across the nation and around the globe, we are refining our measurement techniques and modeling approaches to study interrelated biological processes such as metabolism and immune responses in cancer. Our current efforts with functional imaging may help to address this challenging problem. Another major challenge that lies ahead will be to make our measurement techniques and models accessible to the entire scientific and medical community so that they can be implemented for any patient, anywhere. We are developing partnerships with industry to help realize this goal.
It can be expected that this physics-driven effort will help oncologists determine more effective drug treatment strategies that individualize the amount, frequency and delivery platform of drugs, and assess the need for ancillary non-drug-based treatments. In this manner, we hope to improve the outcomes of patients with cancer.
Vittorio Cristini, Ph.D.
UTHealth Department of Nanomedicine and Biomedical Engineering
Center for Advanced Biomedical Imaging (CABI) – building, Room 3SCR6.4644
1881 East Road, Houston, TX 77054, USA
Website: https://med.uth.edu/nbme/faculty/vittorio-cristini/
ISI Highly-Cited Researchers in Mathematics 2014: http://highlycited.com
Google scholar: http://scholar.google.com/citations?user=uwl5tw0AAAAJ&hl=en&oi=ao
Phone: +1 713-486-2315
Fax: +1 713-796-9697
E-mail: Vittorio.Cristini@uth.tmc.edu
Additional resources:
http://www.nsf.gov/funding/pgm_summ.jsp?pims_id=6673
http://www.internationalinnovation.com/taking-cancer-out-of-the-equation/