Machine Learning methods have received significant attention in the past few years as a game changer for product development and Climate Services
Machine Learning methods, as part of the larger field of Artificial Intelligence, have received much attention in the past few years as a game changer for product development in both business and research. To many, such approaches are a black box, but they also are rapidly replacing many traditional concepts and methods. In fact, several Machine Learning applications show that there is a large benefit in pursuing such options. With the increasing development of very large environmental observational datasets and model outputs, as well as computational capacities that are currently available, data-driven approaches are increasingly providing solutions for solving environmental problems. This is also true for the field of climate science and climate service development.
At GERICS, we are developing and planning several data-driven and Machine Learning applications that have a huge potential to improve the understanding of the implications of climate change for society. It can also help to bridge the divide between vast quantities of climate data, projections of future environmental conditions and simulation of impacts to assets and humans, by adding socio-economic data.
There are broadly three fields in Climate Service development where Machine Learning can be applied (Figure 1). These are: in climate modelling, in the assessment and post-processing of climate hazard data, and in the assessment of climate impacts and adaptation assessment. All these lines will help GERICS to develop new services for end-users to better assess risks and the benefits of adaptation measures and strategies.
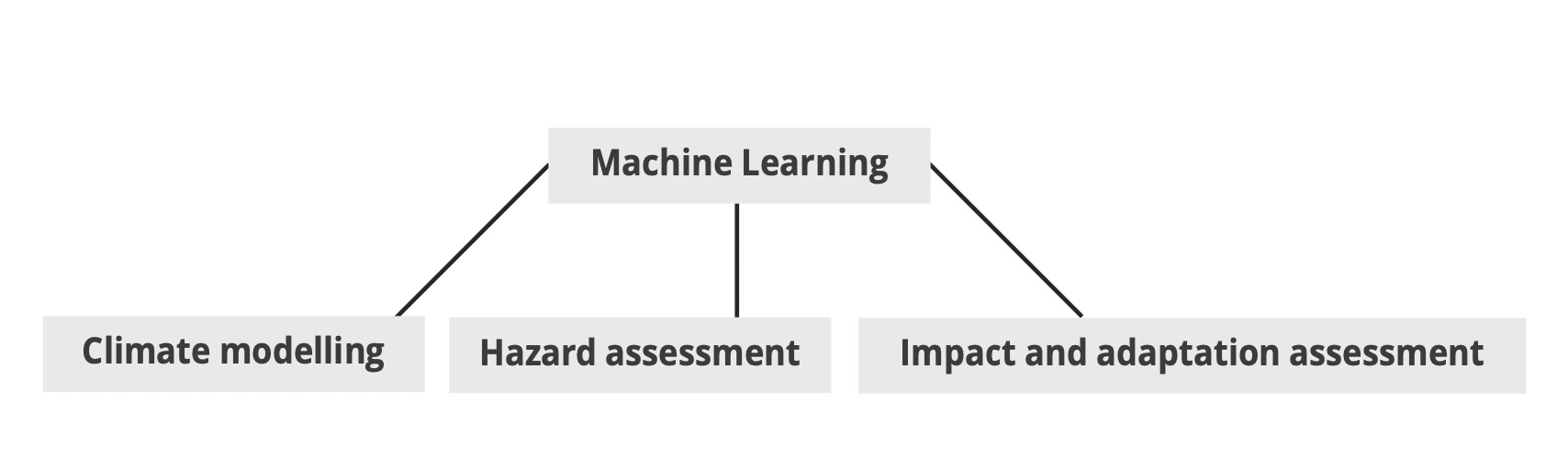
Climate modelling and Machine Learning algorithms
In climate modelling, Machine Learning algorithms can replace numerical equations of physical processes, replace parametrization schemes, or emulate certain processes or even entire models.
Especially relevant for the work of GERICS is the potential to create regional climate information based on deep learning. For example, Baño-Medina et al. (2022) uses convolutional neural networks trained on regional climate model data. In this way, information on temperature and rainfall can be reproduced at the regional scale. This approach saves an enormous amount of computational capacity. It can also speed up the process of developing new regional climate information when new global climate projections become available.
Further efforts focussed on specific climate extremes will substantially improve our capabilities of modelling impacts and help to fill the need for actionable information in the field of Climate Services.
Hazard assessment
GERICS works mostly at the regional to local scale and in urban areas. This often leads to situations where too few weather observations exist (for instance, in dense urban spaces), or when such observations are largely lacking (such as in developing countries). By merging several data sources and types of information, Machine Learning algorithms can describe patterns and interpolate and improve weather and climate condition estimates at the local scale. For instance, random forest models can be applied to predict relative humidity and temperatures at the local scale, thereby greatly improving the spatial resolution of available observational datasets (Nikolaou et al. 2023)
Also, Machine Learning can substantially improve regional simulations and forecasts by post-processing climate information. As we have recently demonstrated, Machine Learning algorithms help to improve rainfall forecasts using observational data (Pakdaman et al. 2022). This improves the usefulness of water resources planning.
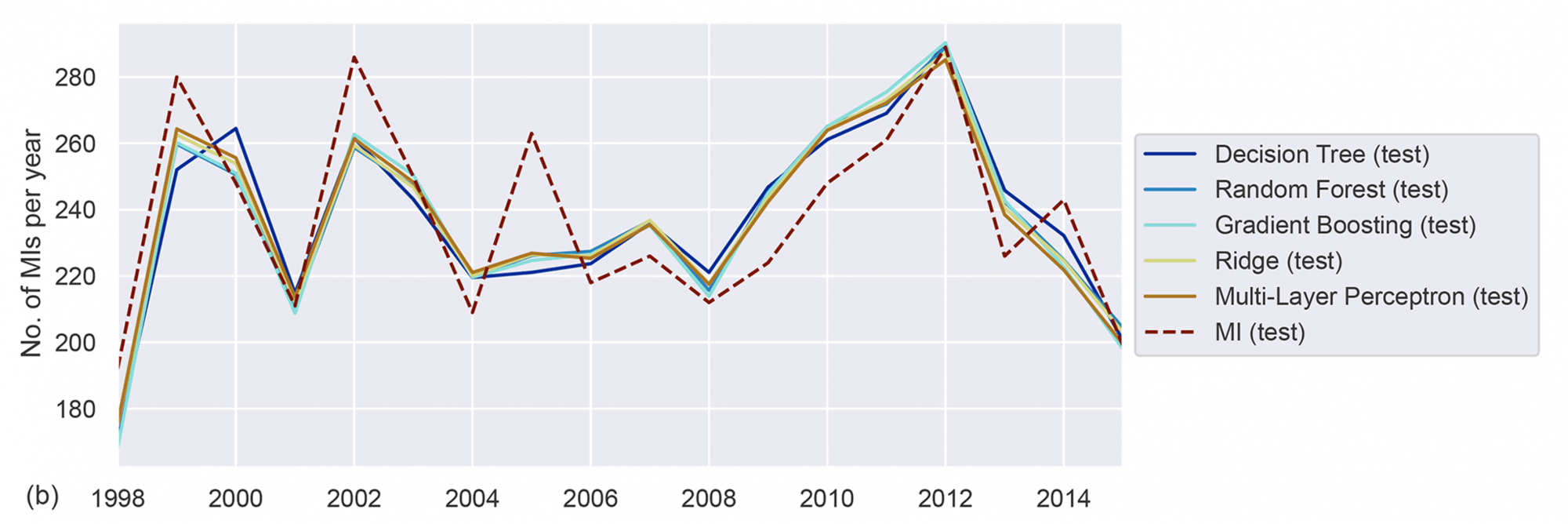
Impact and adaptation assessment
While changes in climatic hazards are often reasonably constrained, the impact or vulnerability models that are used to quantify risks and adaptation are still relatively simplistic. Machine Learning methods can be exploited to help bridge the divide between vast quantities of data produced in large-scale Earth System Models and local-scale socio-economic processes.
GERICS has already been active in developing Machine Learning models for flood and wind damage, where the precision is improved (Wagenaar et al.2021). Also, transferring to other geographic locations and extreme events, where exposure, hazard, and vulnerability characterises differ, has become much easier. Moreover, the methods also show that it is possible to avoid biases and apply the impact models to more extreme events that are only partially captured in the training data. This is particularly interesting when climate change will lead to more extreme events happening in the study locations.
Other applications that GERICS is working on include modelling health effects that are modelled with large accuracy based on data on health outcomes, climate, air pollution, vegetation, and demography. For instance, for the occurrence of myocardial infarction cases (Figure 2). This application allows simulation of the influence of several environmental and socio-economic variables, raising the potential to use these for more precise analysis and evaluation of policy measures.
References
-
- Baño-Medina, J., Manzanas, R., Cimadevilla, E., Fernández, J., González-Abad, J., Cofiño, A.S. & Gutiérrez, J.M. (2022). Downscaling multi-model climate projection ensembles with deep learning (DeepESD): contribution to CORDEX EUR-44. Geoscientific Model Development, 15, 6747- 6758. https://doi.org/10.5194/gmd-15-6747-2022
- “Nikolaou, N., Bouwer, L.M., Dallavalle, M., Valizadeh, M., Stafoggia, M., Peters, A., Wolf, K. & Schneider, A. (2023). Improved daily estimates of relative humidity at high resolution across Germany: a Random Forest approach. Environmental Research, 238, 117173. https://doi.org/10.1016/
- Marien, L., Valizadeh, M., Zu Castell, W., Nam, C., Rechid, D., Schneider, A., Meisinger, C., Linseisen, J., Wolf, K. & Bouwer, L.M. (2022). Machine learning models to predict myocardial infarctions from past climatic and environmental conditions. Natural Hazards and Earth System Sciences, 22(9), 3015-3039. https://doi.org/10.5194/nhess-22-3015-2022
- Pakdaman, M., Babaeian, I. & Bouwer, L.M. (2022). Improved monthly and seasonal multi-model ensemble precipitation forecasts in Southwest Asia using Machine Learning algorithms. Water, 14(17), 2632. https://doi.org/10.3390/w14172632
-
Wagenaar, D., Hermawan, T., Van den Homberg, M.J.C., Aerts, J.C.J.H., Kreibich, H., De Moel, H. & Bouwer, L.M. (2021). Improved transferability of multi-variable damage models through sample selection bias correction. Risk Analysis, 41(1), 37-55. https://doi.org/10.1111/risa.13575
Contributor Details
More About Stakeholder
-
Climate Service Center Germany (GERICS)
The Climate Service Center Germany (GERICS) was initiated by the German Federal Government in 2009 as a fundamental part of the German hightech-strategy for climate protection. Since June 2014, GERICS has been a scientific organizational entity of Helmholtz-Zentrum hereon GmbH. The director of GERICS is meteorologist and climate scientist Prof. Dr. Daniela Jacob. Mission […]